Effects of State Action on Health: a regionalized analysis of Brazil / Efeitos da ação do Estado na saúde: uma análise regionalizada do Brasil
Texto
Imagem
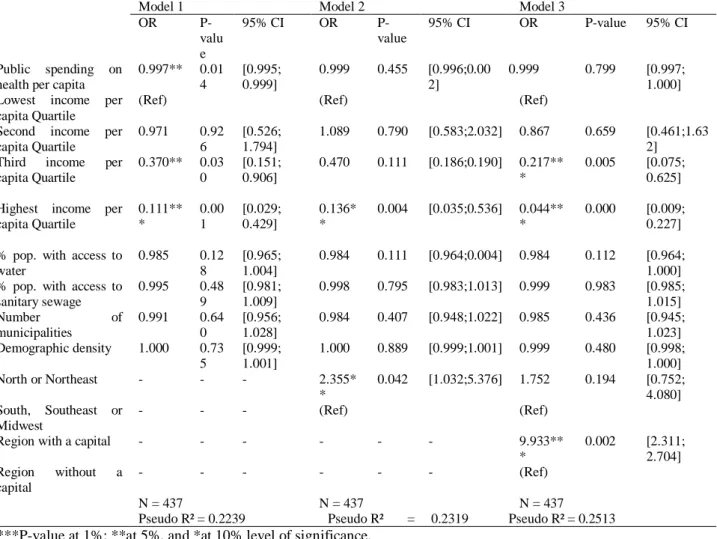
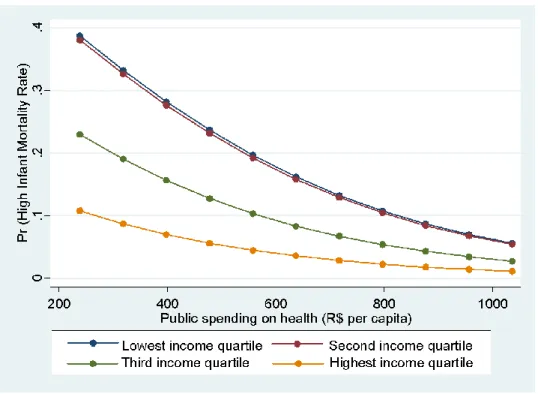
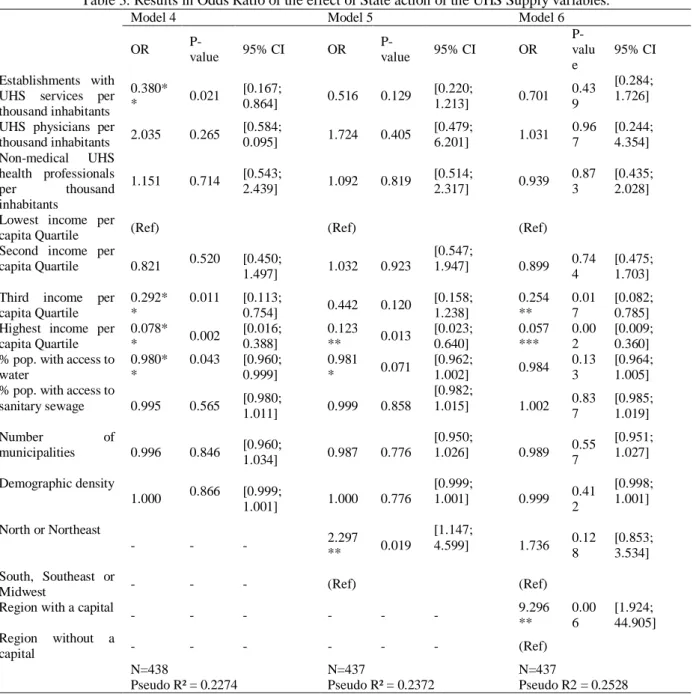

Documentos relacionados
Assim, o objetivo deste estudo foi comparar a epidemiologia espaço-temporal da mortalidade por DTA na 16ª Regional de Saúde/RS, no estado do Rio Grande do Sul e no Brasil,
É preciso lembrar que a ausência de sintomas típicos não exclui o diagnóstico da DRGE, uma vez que outras manifestações relacionadas com o refluxo gastroesofágico têm sido
Devido ao condicionamento estrutural da camada de carvão Barro Branco nesta área, pode-se constatar que bocas de mina que serão tamponadas constituem exutórios para as águas
2.4 Reunião às 17h30: Carlos Borges da Silva Junior, Marilyn Mafra Klamt, Charlene Bezerra dos Santos, Daniele Souza Freitas Pacheco e Isabel Maria
f) 1 (um) Centro de Reabilitação – CER tipo II, com especialidade em reabilitação física e auditiva no município de Fortaleza. g) 1 (um) Centro de Reabilitação – CER tipo
No quadro SELECIONE UMA EMPRESA, será listado os estabelecimentos matriz e filiais se houver, ao selecionar a entidade, deve preencher os campos: ESTOQUE INICIAL, ESTOQUE
em cor de amizade e jardim. Meus amigos estão construindo um mundo aéreo para mim. Mãos tão frágeis levantam muros, corpos voantes transportam ruas, todos num silêncio conjunto
Tomando como fundamento as conclusões até aqui realizadas, insta retomar a problemática central da presente pesquisa que, partindo da hipótese de que os Conselhos