This new approach to the description of the singing voice provides information that complements information related to voice timbre. We propose to improve the recognition rate of vocal partials by exploiting the harmonic nature of the singing voice.
Features transformation and selection
This type of method compresses the information about the original feature into a smaller set of features. To optimize the classification performance, features can be selected according to their capacity to separate the classes of the problem.
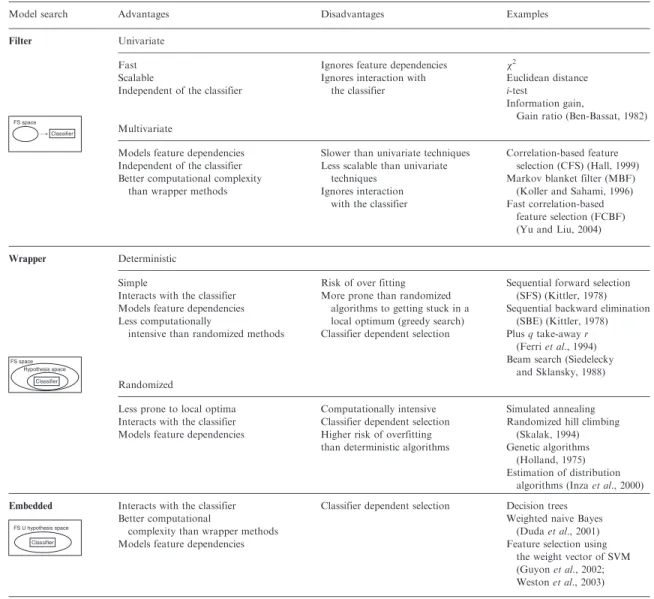
Classifiers architecture
Generative approach: GMM
The density modeling accuracy obtained by an M-component GMM with a full covariance matrix can be equal to a GMM with a higher-order diagonal covariance matrix (M' . > M). Fitting a GMM is not straightforward: the number of mixtures, the training method, and the choice of covariance matrix type can be optimized by a cross-validation procedure on the training data set (but this significantly increases the risk of obtaining overfitted models).
Discriminative approach: SVM
To calculate the pseudo posterior probability for each problem class, the proposed methods, [WLW04] and [GTA08] can be applied. SVM appears to be less sensitive than other classification methods to feature space selection (feature set selection and transformation).
Instance-based approach: k-NN
SUPERVISED FURNITURE RECOGNITION 21 – The main advantage of the k-NN algorithm is the simplicity of interpretation and imple-. The computational cost increases with the dimension of the feature space and the size of the training set.
Performance of classification
Measure of performance
This representation shows how the number of samples of the positive class correctly classified (tp) varies. The classifier whose curve is closer to the top left corner of the plot performs better.

Comparison of classification performances
First, the combination of information can be performed at any stage of the classification process. However, in many situations it is only possible to combine the decisions of the classifiers.
Levels of combination
Feature Combination Combination of descriptors, or "feature fusion", consists of combining different descriptors of the same pattern to form a unique descriptor: D(z) =[Ml=1Dl(z). A significant effect of this type of sampling is the reduction of the noise that can be created by errors in the description.
Schemes for combination of classifiers decisions
Parallel combination
As shown in Section 1.2, these membership measures can be a (pseudo)-posterior probability (GMM) or a distance (SVM, kNN). In this case, each classifier produces an estimate of the same membership measures and they can be combined directly without risk.
Sequential combination
The different decisions are obtained by changing the parameters of the classifier (eg the number k or the distance for kNN, the kernel for SVM, or the number of Gaussians for GMM, etc.). A specific feature set can be used by each classifier at each step of the process.
Trainable .vs. non-trainable combiners
We present in Section 1 the basic principles of vocal production on which the interpretability of the source filter and sinusoidal model relies. In many cases, however, signals from the singing voice are heard as distinctly different from speech.

Vocal production
Some of the elements that make singing so different from speech are presented in Section 1.2 following an introduction, in Section 1.1, of the basics of vocal production involved in the production of speech and singing. A comprehensive description of each step of song production can be found in the extensive works of Sundberg [SR90] and Titze [TM98].
Some specificities of the singing production
- Formants tuning
- The singing formant
- Vocal vibrato
- Portamento and Legato
The studies of vibrato speed measurement show that the speed of the vocal vibrato varies between 5 and 8 Hz. The frequency modulation of the vocal vibrato is a quasi sinusoidal modulation with small fluctuations [Sea31].

Source-filter model
Model description
These studies consider the voice source to be related to the qualities of the vocal folds (length, mass, tension), and they suggest that the filter is related to the physical shape of the vocal tract. The contribution from the source and the filter are generally separated using inverse filter techniques, where h(t) is given by the estimation of the spectral envelope of the|X(!)|amplitude spectrum of the signal.
Estimation of the vocal transfer function
The true envelope estimate is based on iterative cepstral smoothing of the log amplitude spectrum. Let X(!) be the spectrum of the signal and Vi(!) the cepstral representation at stepi (i.e. the Fourier transform of the filtered cepstrum).
Harmonic sinusoidal model
Model description
With this procedure, the valley between the peaks is filled by the filtered cepstral representation and the estimated envelope will gradually grow until the peaks are covered.
Sinusoidal model parameters estimation
The off value is given by the position of the highest peak in the spectrum. The score is given by the value of the field where the spectrum is largest.
Intonative model
Model description
In practice, the speed of the vibrato remains almost constant throughout the duration of the note, but the amplitude can vary considerably. To allow a more flexible model of vibrato, we propose to describe the amplitude of the modulation with an exponential.
Model parameters estimation
Taking these points into account, we suggest modeling the frequency trajectory of the partial as the sum of the periodic modulation xf(t) plus the slow continuous variation df(t). Thus, the modulation parameters (rate, amplitude, and phase) can be estimated using any of the methods presented in Section 2.2.2.
Model evaluation
We evaluate the performance of the method for estimating the vibrato parameters on S1 and S2. The performance of the estimation of all parameters of the model is evaluated on the signals of S3.
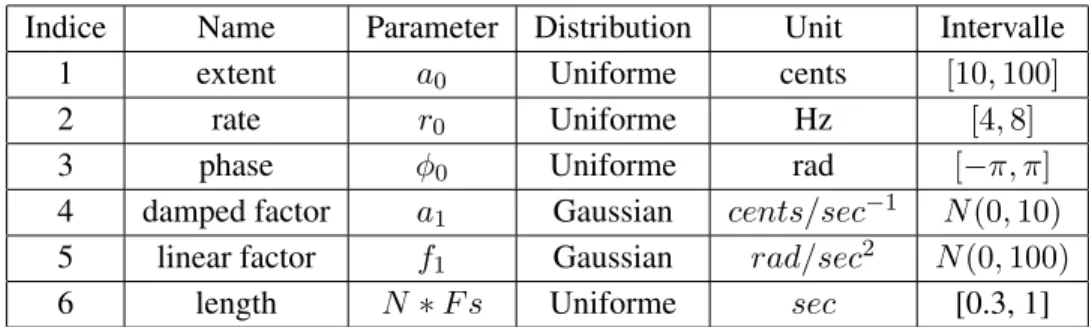
Relation between intonative and source-filter model
Estimation of the formant position from a cycle of frequency mod-
The frequencies of the partial cross the formant: In this case, the frequency and bandwidth of the formant can be easily derived, as illustrated in Figure 3.26. The frequency of the formant is simply given by the position of the peak with maximum amplitude.
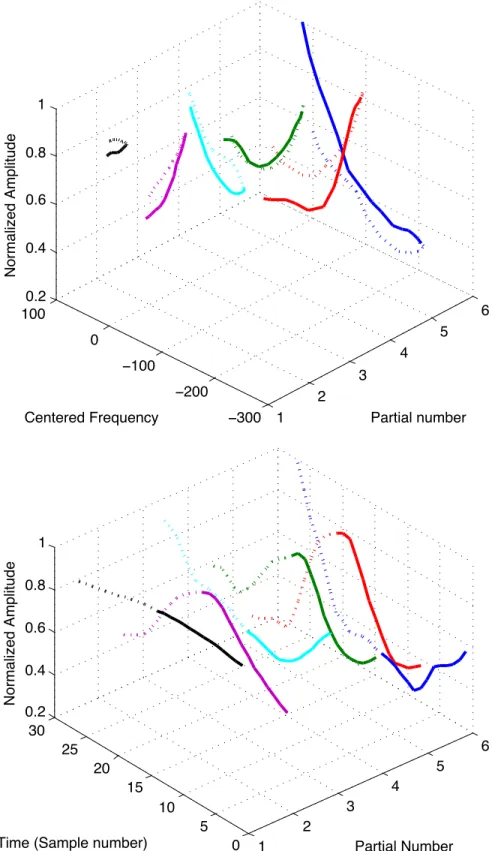
Timbral features
Mel Frequency Cepstral Coefficients As explained in Section 2.1.2, the second main idea we explored for spectral envelope estimation is based on the cepstrum. In the cepstral representation of sound (see Equation 3.5), the envelope is estimated using the low cepstral frequencies.
Intonative features
They depend on the technique (vibrato speed at a given average frequency) and the vocal tract (ratio of AM and FM frequencies for a given average frequency) of the singer. The coefficients given by the linear prediction can be used directly as a compact representation of the singer's vocal tract.
Singing voice detection
In this chapter we investigate the following points: 1) locating the vocal parts of a song and 2) tracking the content produced by the singing voice within the signal of a song. To obtain an accurate transcription or extraction of the vocals, it is necessary to retrieve within the signals the elements produced by the voice.
Singing voice tracking
Each of the four problems mentioned above is complex and each of them could be the subject of a dissertation. Therefore, it is clear that the reviews presented here are not exhaustive and are given to give a general idea of the problems associated with extracting information from the singing voice of a song.
Singing voice localization
The study shows that performance variation is greatly influenced by feature selection. The idea of TRACT is also related to the harmonic character of the singing voice.
Instrument identification
Solo instrument identification
Finally, the regions where the energy of the filtered signal is higher than a certain threshold are classified as vocal. The use of the taxonomy of musical instruments was also investigated by Eronen and Klapuri [EK00] on the same dataset as [MK98].
Multiple instruments recognition in polyphonic recordings
A comprehensive review of features and statistical classifiers used for musical instrument recognition as proposed by Herrera [HBPD03]. It is also suggested to subtract the values corresponding to the first instrument, and to keep the remaining values for the recognition of the second instrument.
Singing voice extraction using source separation approach
Blind Source Separation (BBS) approaches
In their approach, the STFT of the signal is calculated and analyzed to initialize the NMF input (i.e. the non-negative matrix). This phase is followed by a manual selection of the spectral bases associated with the singing voice.
Statistical Modeling approaches
This estimate of the melody is then processed by the Viterbi algorithm to improve the quality of the melody tracking. The results of the Viterbi algorithm can be used to reestimate the singing source as proposed in [DRD09].
Computational Auditory Scene Analysis (CASA) approaches
The vocal parts of the song are broken down into time-frequency (T-F) units (the time frame and frequency channels given by the auditory filter bank). On the other hand, many singing voice extraction approaches are based on the estimation of the sung melody.
Singing melody transcription
They compare the effectiveness of their approach with the effectiveness of the method proposed by Goto [Got04]. The method proposed by Ryynanen and Klapuri [RK06] estimates multiple f0s and note onsets (accent detector) at each frame of the signal.
Description of the proposed approach to localize vocal segments
- Step 1 - Partial tracking and segmentation
- Step 2 - Extraction of features
- Step 3 - Selection of vocal partials
- Step 4 - Formation of vocal segments
Applied to the frequency curve (x(t) = fp(t)), this model estimates the parameters of portamento and vibrato. The same model applied to the amplitude trajectory (x(t) =ap(t)) estimates the tremolo parameters.
Evaluation
Data set
All songs of the data set were manually annotated by the same person to provide a reference for the position of vocal segments. In the following, 58 songs are used for the training and the evaluation is done on the 32 remaining songs.
Results
The goal of the method is to get all the parts that correspond to the singing voice in a mix. The main purpose of the method is to get all the parts produced by the sound in a mixture.
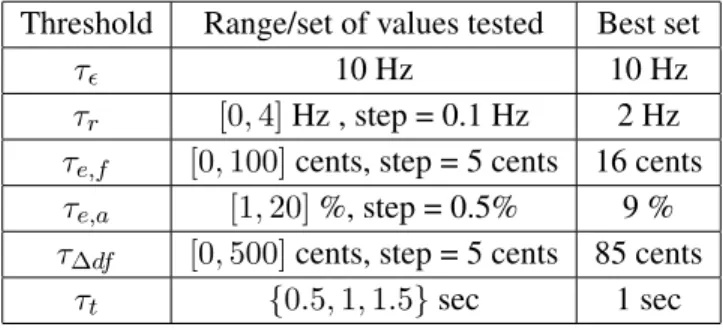
Description of the method to group harmonically related partials
- Theoretical fundaments
- Step 1 - Measure of similarity between partials
- Step 2 - Distance matrix
- Step 3 - Grouping harmonically related partials by clustering
The main idea of the method is to define a similarity measure between partials based on the parameters vibrato and portamento. We define a first criterion based on the length of the joint support of the two parties.
Evaluation of clusters of harmonic partials
Data set
In Figure 4.3 we plot the result of the proposed method, calculated on a real signal with three sources (voice and piano), with the partial parts of each source extracted on separate tracks. Before explaining the details of the basic frequency estimation method and its application to multi-pitch estimation, we first evaluate the method's ability to group harmonically related partials.
Measure for cluster evaluation
For each song, one or two instrumental tracks are chosen so that there are the same number of instrumental parts as vocal parts. Finally, the balanced sets of passages for each song are merged into a global set of passages.
Results
However, clusters of vocal partials cannot cover multiple note events due to the first comparison function given in equation (4.14). In the following sections, we apply the clustering method to particles obtained from a mixture of instruments.
Application to singing voice detection
Data set
SUGGESTED APPROACH TO CARRYING HARMONIC CONTENT OF THE VOCAL VOICE95 the instruments considered in the accompaniment are polyphonic instruments which makes the task even more complicated. We also note that the cluster purity measure does not consider the problem of note events.
Results
Furthermore, the clusters of harmonic partials are powerful for two other applications: the separation of the singing voice and the transcription of the vocal melody. In the next section, we present a method to transcribe the vocal melody based on the analysis of the partial clusters.
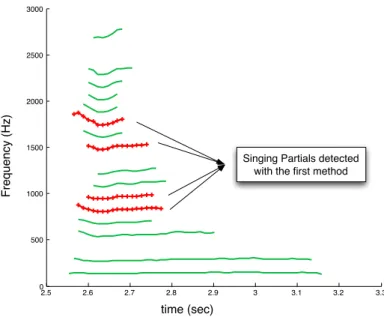
Application to multi-pitch and sung melody transcription
- Method to estimate the f 0 of a cluster
- Data set
- Measure
- Results
On the a cappella recordings, the fundamental frequency of the vocal melody is estimated using the YIN algorithm. The vocal melody is determined by the fundamental frequency of the clusters classified as vocal clusters.
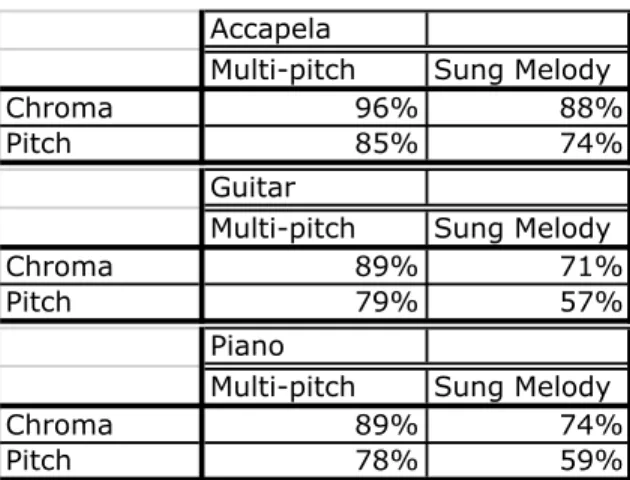
Artist identification
The goal of singer identification (SID) is to retrieve the name of the singer performing a given song. Finally, a query song is tagged with the artist name of the most similar song.
Singer identification
In [ME05], Mandel and Ellis propose to represent an artist with a set of song patterns obtained for each song of the artist. The purpose of the study is to assess the reliability of singer models obtained in accompanied vocals.
Perceptual recognition of singers
According to the results of the studies presented in Section 2.3, it is likely that these features (vibrato parameters, the ratio between vibrato and tremolo rates, and portamento parameters calculated at the note transition) convey information about the identity of the singer. In Section 3.1, after discussing the complementary aspect of the two types of features, we give the details of the proposed singer identification approach.
Description of the proposed approach based on the combination of timbral
Sound descriptions complementarity
The main idea of the proposed method is to increase the performance of singer identification systems by combining timbre and intonation features. The purpose of the proposed method is to increase the singer identification performance by combining complementary information about the signal to be classified.
Combining decisions obtained with each sound description
In the present case, a query sample is given as input to the classification system based on timbral features. The number of classes remaining at the end of the first phase is dynamically selected.
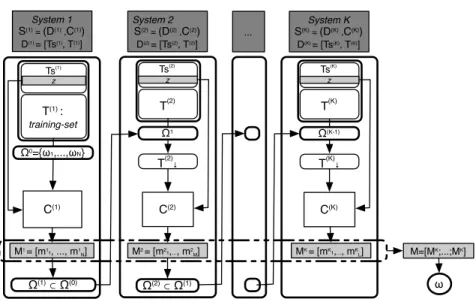
Evaluation of the combination method
Data sets
We report in Tab.5.2 the results obtained with LYR and in Tab.5.3 the results obtained with POP. Finally, the performances achieved with the combination are reported at the intersection of the two systems used.
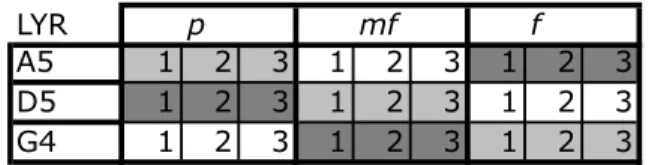
Description of the proposed approach
The study shows that singer identification was affected when the phonemes in the query samples were different from the phonemes used to train the singer models. It is justified to wonder, with respect to the conduct of these studies, whether spectral features can capture information about the singer's voice when extracted on accompanied vocals.
Evaluation of the features of robustness against intra-song and inter-song vari-
Results for intra-song variations on a cappella recordings
To evaluate the performance of features against height variation, we placed all samples with the same pitch in the same fold to create 3 folds. We report in Fig.5.7, the results of the same experiments for each category of singers.
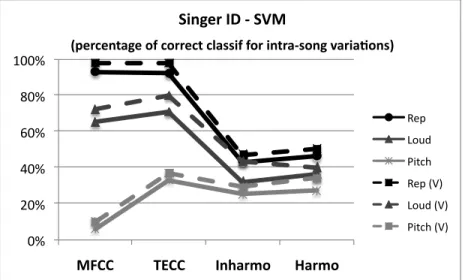
Results for inter-song variations
The improvement lies in the additional information that the two descriptions of the singing voice provide. The results obtained in this research prove that the vibrato is an important characteristic of the singing voice.
