In this thesis, we evaluate how vulnerable existing 3D and 2D face recognition systems are to fake mask attacks. At the beginning, we show how vulnerable existing 3D and 2D face recognition systems are to fake mask attacks.
Original Contributions
To our knowledge, the impact of 3D mask attacks on both 2D and 3D face recognition systems was first analyzed in our study [74]. For face change analysis, since there is no publicly available 3D plastic surgery database to simulate face changes, we have prepared a 2D+3D nose change database, which allows analyzing the impact of face changes on both 2D and 3D face recognition.
Outline
Biometrics
On the other hand, in identification mode, all biometric references in the gallery are examined and the one with the best match score indicates the class of the input. In verification mode, if the match score is above a certain threshold, the identity claim is accepted.
Face Recognition
An overview of the literature on facial recognition spoofing is presented, including the impact analysis and countermeasures. This is why in the second part we describe disguise variations and then a review of the literature on disguise variations in facial recognition, including the impact analysis and countermeasures, is presented.
Spoofing in Face Recognition
In our study [74], we analyzed how well the spoofing performances of the masks used in our studies are. Therefore, it is not possible to learn the spoofing performance of the used masks (shown in Figure 2.2) in 3D face recognition from their study.
![Figure 2.1: An example photograph attack for spoofing purposes. Figure is taken from [64].](https://thumb-eu.123doks.com/thumbv2/1bibliocom/461478.67942/33.892.258.652.323.556/figure-example-photograph-attack-spoofing-purposes-figure-taken.webp)
Disguise Variations in Face Recognition
Facial Alterations and Countermeasures
Unfortunately, for face recognition algorithms, the accuracy can vary greatly depending on the difficulty of the database. This leads to an additional decrease in the performances which obscures the true measurement of the plastic surgery effect.
![Figure 2.3 shows an example from the plastic surgery database explained in [127].](https://thumb-eu.123doks.com/thumbv2/1bibliocom/461478.67942/38.892.203.675.357.798/figure-shows-example-plastic-surgery-database-explained.webp)
Facial Makeup and Countermeasures
A rare study of the facial cosmetics challenge in face recognition can be found in [29]. The authors state that facial cosmetics have an impact on the performance of facial recognition systems.
Facial Accessories (Occlusions) and Countermeasures
In [128], the impact of masking variations is evaluated on several face recognition techniques using a database. We perform benchmark evaluations on the proposed database using a variety of basic face recognition methods.
![Figure 2.5: Example of images for occlusion from the AR Face Database [91].](https://thumb-eu.123doks.com/thumbv2/1bibliocom/461478.67942/41.892.240.678.180.415/figure-example-images-occlusion-ar-face-database.webp)
Conclusions
The proposed KinectFaceDB is compared with a widely used high-quality 3D face database (i.e., the FRGC database) to show the effects of 3D data quality in the context of facial biometrics. The results show that the approach is competitive with other existing methods tested on the same database.
The Photograph Database
For a precise comparison with the existing methods in [131], the same database is selected and experiments are performed with the same split train set. In the NUAA database [ 33 ], 6 subjects out of 9 subjects do not appear in the live human case set, and 6 subjects out of 15 subjects do not appear in the photo case set.
The Proposed Approach
Pre-Processing
This indicates that in our study, the subject shown in the test set may not appear in the train set, which makes the detection of photo deception more challenging. The positive effect of applying DoG filtering as a pre-processing step in the proposed approach is also verified in Section 3.4.
Feature Extraction
From equation (3.10) it becomes clear that the distance between two histograms consists of two parts. In equation (3.12), N is the number of images in the model set and xi is the distance between test sample and model sample.
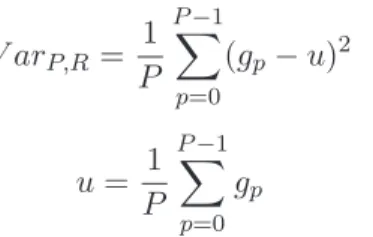
Experiments and Analysis
Test 1: Results under Illumination Change
According to the results in Figure 3.4, it is clear that the proposed approach is quite competitive with the existing techniques, even better compared to most of the results shown in this figure. The comments from figure 3.4 are; initially, the proposed approach provides better results compared to the results obtained by applying SLR classification to all input feature types.

Test 2: Effect of DoG Filtering in the Proposed Approach
For example, for techniques based on LTVu and LTVfused functions, the image must be decomposed into illumination and reflectance components; while in the proposed approach the method is applied directly on the image. Therefore, we can say that the proposed method can be considered to provide a sufficient detection accuracy of 88.03% with the advantages of lower computational complexity, robustness to changes in illumination and rotation.
Conclusion
To the best of our knowledge, the impact of mask spoofing on facial recognition was first analyzed in our study [74]. This chapter provides new results by demonstrating the impact of mask attacks on 2D and 3D facial recognition systems.
The Mask Database
The purpose of the study [74] was not to propose a new face recognition method, but instead to show how vulnerable the selected baseline systems are to spoofing mask attacks. For DB-m, an average of 10 scans were taken of each subject wearing either his own mask or masks of the other subjects appearing in the same database.
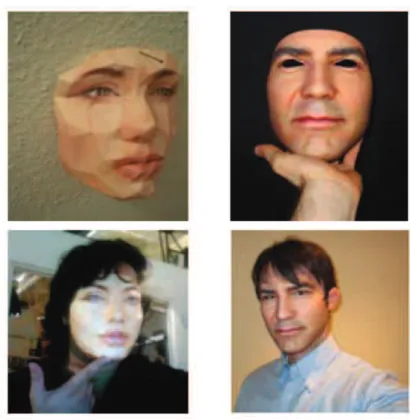
The Selected Face Recognition Systems
Pre-Processing
For 2D face recognition, the texture images in the mask database are cut as shown in Figure 4.2 and rescaled to 64×64 images. In the next chapter, we will show how the countermeasure improves performance in the presence of masking attacks by evaluating the performance of these systems with/without countermeasures.
Face Recognition Systems
In this chapter, we aim to show how vulnerable existing systems are to mask spoofing attacks by evaluating the performance of selected systems with and without attacks. As explained in the previous chapter, in equation (3.9), the value of U is the number of spatial transitions (0/1 bit changes) in the sample.
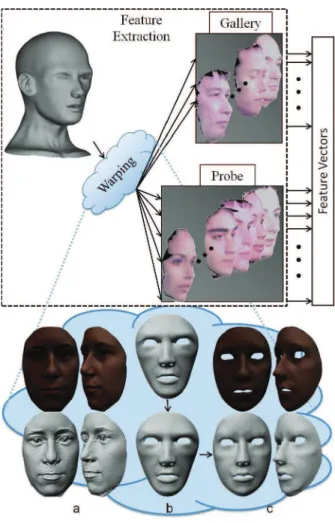
Experiments and Analysis
Scans of subjects in the mask database were taken with a high-quality laser scanner (MORPHO technology). Therefore, the scan quality in FRGC is quite similar to the scan quality in our mask database.

Conclusions
In this chapter, countermeasure techniques are proposed to protect face recognition systems from masking attacks. In this chapter, we will explain our countermeasure techniques proposed for detecting the mask attacks to protect face recognition systems against mask fraud.
Countermeasure Techniques Against Mask Attacks
Techniques used Inside the Proposed Countermeasures
In the previous chapter, a brief information on the mask database used in our studies is given. For the countermeasures proposed in this study, the image is decomposed into reflectance and luminance components using the variational retinex algorithm explained in studies [6, 67].
![Figure 5.1: Example from the mask database which is created by [98] (a) The real face with texture, the reflectance image and the illumination image of the real face (b) Same images associated with the mask of the same person.](https://thumb-eu.123doks.com/thumbv2/1bibliocom/461478.67942/71.892.171.736.276.596/figure-example-database-created-texture-reflectance-illumination-associated.webp)
The Proposed Countermeasures
The captured image of the mask can be visually very similar to the image captured from a live face (for example, the texture images in the second column of Figure 4-2). The 3D shape of a high-quality mask also closely resembles the 3D shape of the corresponding real face (for example, the 3D scans in the third column of Figure 4-2).

Experiments and Analysis
Stand-Alone Classification Performances of the Countermea-
After evaluating the performances of the single countermeasures, we analyze the performances for the merger scenarios. Both score and feature level fusion of the countermeasures improve the performance compared to using single countermeasure.

Integration of the Countermeasure to 3D Face Recognition
The countermeasure performance is observed to be better at FFR = 5% compared to the cases at FFR = 1% and 10%. Finally, the inclusion of the countermeasure improves the results of the 3D FR system under attacks, while degrading the base performance of the system when not facing an attack (pink curve compared to blue curve).
Conclusions
Three of the proposed countermeasures use 2D data (texture images), and the remaining one uses 3D data (depth images) as input. For this reason, the impact of the face changes on 3D algorithms should also be investigated.
![Figure 6.1: Examples of nose alterations with before (upper row) and after (lower row) photos: (a) plastic surgery [133] (b) latex appliance [120] (c) makeup using wax [19]](https://thumb-eu.123doks.com/thumbv2/1bibliocom/461478.67942/84.892.215.654.247.629/figure-examples-alterations-photos-plastic-surgery-appliance-makeup.webp)
Simulating Nose Alterations
In this way, a 2D+3D database of nasal changes is obtained and since the conditions and subjects are identical for the original and simulated database, it is possible to measure the exact impact of the applied changes. The original FRGC v1.0 database consists of 943 multimodal samples from 275 subjects and the simulated 3D database is of the same size.
Experiments and Analysis
Impact on 2D Face Recognition
Verification rates at 0.001 FAR and ROC curves for all three algorithms are given in Table 6.2 and Figure 6.4, respectively. According to the results in Table 6.1 and Table 6.2, the best performance is obtained using the LBP method for identification and verification with a distinct difference.
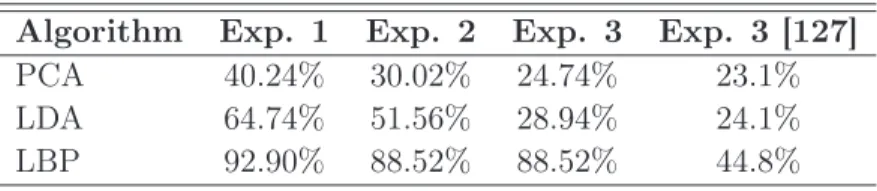
Impact on 3D Face Recognition
Likewise, analysis regarding verification rates reveals that LDA and WP are less affected by nasal changes.
Conclusion
It is used to evaluate the performance of facial recognition algorithms in the presence of nasal changes. In the next chapter, we propose a block-based facial recognition approach that is robust to nasal changes.
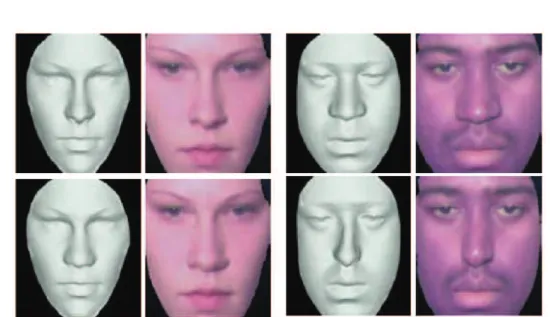
Block Based Face Recognition Approach Robust to Nose Alterations 74
Evaluation on 2D Face Recognition
From Tables 7.3 and 7.4, it is also clear that using the proposed approach, the performance degradation due to face changes is lower compared to the results obtained with the holistic approach for all three techniques. This proves that the proposed approach improves the recognition performance and provides stronger results for face changes compared to the holistic approach.
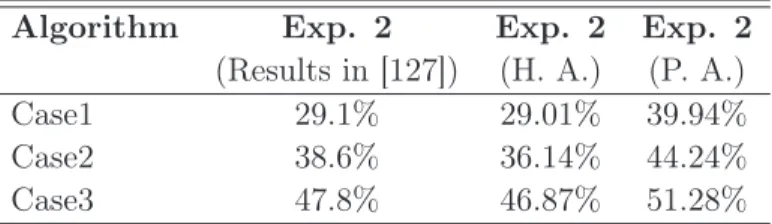
Evaluation on 3D Face Recognition
On the other hand, there is also an increase in the performance for LBP with the proposed approach, but it is less compared to PCA and LDA. Similar to analysis on texture images, the proposed approach again offers both better performances and also more robust results compared to the holistic approach.
Conclusion
In this study, preliminary tests are performed regarding the impact of facial cosmetics on facial recognition. In section 8.4, preliminary tests are presented regarding the impact of facial cosmetics on facial recognition.
![Figure 8.1: Impact of Facial Cosmetics on the Perception of a Face. This figure is taken from [138].](https://thumb-eu.123doks.com/thumbv2/1bibliocom/461478.67942/108.892.252.687.159.374/figure-impact-facial-cosmetics-perception-face-figure-taken.webp)
Facial Cosmetics Database
Specification of the Database
Acquisition of Images
The most used videos are taken from YouTube, some of them are taken from the websites of facial cosmetics companies. To ensure different conditions for reference and test images as described in section 8.2.1, at least two videos are required for each person.
Structure of the Database
Facial Cosmetics
Specification of Facial Cosmetics
Covering skin imperfections and natural shadows; change in skin color, face and nose shape; reduction of gloss. Emphasis on the folds of the eyelids; increase in depth; a change in the perceived size and color of the eyes.
Classification of Applied Facial Cosmetics
If any makeup step on the eye area is lightly applied, this is classified as “light”. Heavy application of make-up causes changes in the size and shape of the lips, which is why we classify them as "heavy".

Evaluation and Results
Test Setup
The facial makeup category as a whole is equal to the highest category of the subareas. However, there is a special case, since the subarea categories contribute disproportionately to the facial makeup category, mouth makeup has little influence on the overall perceived amount of makeup.
Evaluation Results
Compared to the 'slight' and 'heavy' categories, intermediate make-up images present the highest IDR, which is almost as high as the IDR calculated for images where no make-up is applied. The IDR curve for face recognition with intermediate makeup reference images is higher compared to the one resulting from no makeup images used as references.
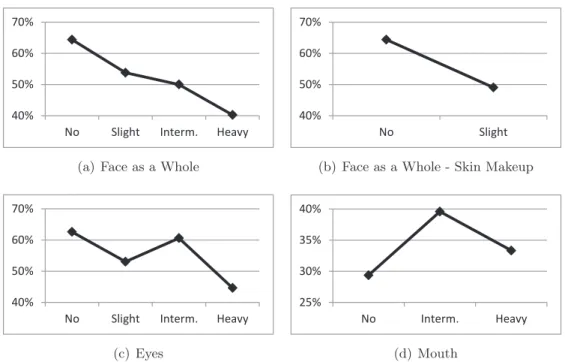
Conclusion
We also compare the Kinect images (from the KinectFaceDB) with the traditional high quality 3D scans (from the FRGC database) in the context of face biometrics, demonstrating the essential needs of the proposed database for face recognition. In this chapter, a standard database (i.e., the KinectFaceDB) is presented to evaluate face recognition algorithms based on the Kinect.
Review of 3D Face Databases
Recently, UMB-DB [27] is proposed for the evaluation of 3D face recognition under occluded conditions. Almost all 3D face databases are also multimodal in the sense that 2D texture images are also provided (except 3D-RMA).

The Kinect Face Database
- Database Structure
- Acquisition Environment
- Acquisition Process
- Post-Processing
- Potential database usages in addition to face recognition
However, due to the intrinsic architecture of the Kinect sensor (where RGB images and depth images are sampled separately from two different cameras with displacement between them), the RGB image and the depth map captured by Kinect are not well aligned. Finally, we store video sequences of aligned RGB and depth frames from the RGB camera and the IR camera using the protocol described in Section 9.3.1.

Benchmark Evaluations
- Baseline Techniques and Settings
- Pre-processing
- Evaluation Protocol
- Evaluation Results
- Fusion of RGB and Depth Face Data
The ROC (receiver operating characteristic) curves of PCA-based method for both 2D- and 2.5D-based face recognition are shown in Figure 9.9. Since the depth map is very smooth, the SIFT-based method is inappropriate for 2.5D-based face recognition.

Data Quality Assessment of KinectFaceDB and FRGC
This phenomenon suggests that a simple but effective depth description using 2.5D depth images is preferred for Kinect-based face recognition compared to methods using sophisticated surface registration based on 3D points. Although the 2.5D/3D face recognition capabilities of Kinect are inferior to a high-quality laser scanner, Kinect's inherent advantages make it a competitive sensor in real-world applications.
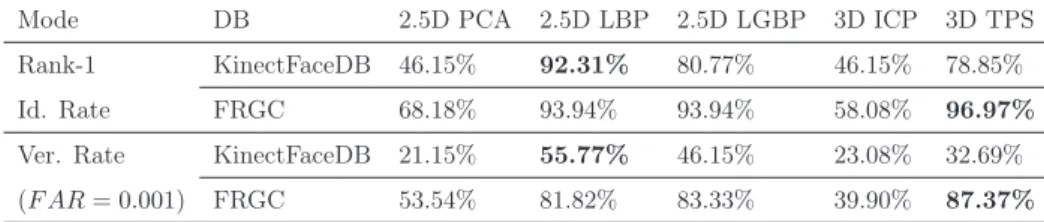
Conclusion
In Chapter 4, we analyzed the impact of masking attacks on both 2D and 3D face recognition in detail. The impact of each of these disguise variations on facial recognition systems is different.
Future Work
Dans le prochain chapitre de la thèse, nous montrons les vulnérabilités des systèmes de reconnaissance faciale au masquage des attaques. Des études récentes montrent que les systèmes de reconnaissance faciale sont vulnérables à ces attaques.
![Figure 11.1: Chaque colonne contient des échantillons de la session 1, session 2 et session 3 de la base de données NUAA [131]](https://thumb-eu.123doks.com/thumbv2/1bibliocom/461478.67942/151.892.258.647.654.953/figure-colonne-contient-échantillons-session-session-session-données.webp)
Summary of Off-the-Shelf 3D Face Databases
Rank-1 Identification Rate for 2D Face Recognition
Rank-1 Identification Rate for 2.5D Face Recognition
Rank-1 Identification Rate for 3D Face Recognition
Fusion of RGB and Depth for Face Recognition Rank-1 Identification
Fusion of RGB and Depth for Face Recognition Verification Rate
KinectFaceDB vs. FRGC