Соколова Елена Григорьевна Российский научно-исследовательский институт искусственного интеллекта Толдова Светлана Юрьевна Филологический факультет МГУ Шаров Сергей Александрович Университет Лидса, Великобритания. Чтобы уменьшить вариативность результатов выбора единицы, мы рассчитываем статистическое обобщение интонации говорящего.
Introduction
System description
Number of phones in the next syllable Vowel name in the current syllable Syllable position from the beginning of the word. Number of syllables in the previous word Word position from the end of the sentence Sentence feature.

Experiments
The purpose of the unit selection algorithm is to select a sequence of elements that minimizes the final cost equation (3). We performed a MOS (Mean Opinion Score) evaluation to assess the naturalness of the synthesized speech.

Conclusions
- Break detection using statistical methods
- Experimental setup
- Results and discussion
- Break placement
- Break durations
- Conclusions and future research
- Введение
- Существующие подходы
- Предлагаемый подход
- Особенности украинской и русской фонетических систем
- Речевые и текстовые данные
- Система распознавания украинской речи
- Экспериментальная система
- Экспериментальные результаты
- Обсуждение результатов
- Заключение
In Table 1, we present the results of automatic pause placement (CART and Random Forest) compared to the results of the "baseline" rule-based algorithm currently implemented in the standard version of the VitalVoice TTS system [14] . The classifier's results also compare well with those reported in the literature.

Литература
- Rule-based approach
- Hybrid approach
- Experiment
- Conclusion
- Query-Based Sentiment Extraction
- Sentiment Classification of User Reviews
- News-Based Opinions Classification
- Official metrics
Part of the next word Word position from the beginning of the sentence Number of syllables in. Proceedings of the International Conference "Dialog 2000" [Komp'iuternaia Lingvistika i Intellektual'nye Tekhnologii: Trudy Mezhdunarodnoi Konferentsii.

R Fmeasure P
- Results Overview
- Review classification task
- News-based opinion classification
- Query-based sentiment extraction
- Conclusions
- Existing approaches
- Lexicon-based method
- Machine learning methods
- Training collections
- Dictionary creation
- Features
- Classification methods
- Experimental results
- Conclusion
For the support vector machines according to the results of [23], the reviews were represented as binary vectors with cosine normalization. Thus, we can conclude that the methods used in the seminar ROMIP2012 are more stable in the face of the increase in the number of classes.

Introduction
Problem specification
Generalizing facts with semantic filters
The main disadvantage of this approach is the need to manually select the terms for the filters. In addition, the quality of the selected terms can be improved by increasing the representativeness and size of the training sample.

Classification methods
Despite rare errors (for example - the term "optimistic" is included in a filter for the negative class), most of the vocabulary is adequate.
Results
It is also clear that in the case of the hybrid model, the regression-based classifier shows better result than SVM. It is worth mentioning that the test set was evaluated by only one expert, which leads to increased bias in the final result.
Results analysis
It turned out that the new classifier showed an improvement in the classification into 3 classes compared to the hybrid system and even the regression method [6] which was the leader among all the systems that participated in the process last year. It follows that the new classifier performs better than the old one, provided the set is not biased.
Possible improvements
Third, by classifying into three classes, the author's opinion should be distinguished from the opinions of external sources ("говорят книга хорошая, но мне не очень понравилас"). When classifying into three classes, this factor is not so critical, and different sources can be assigned equal weight.
Conclusion
- Linguistic rules in sentiment analysis systems
- POLYARNIK system for sentiment classification of socio-political texts
- The POLYARNIK system in ROMIP-2012 news-based opinion classification task
- Testing various rules for news-based sentiment classification
In addition, the study describes several rules that take into account the appearance of irrealis marks (words indicating that a certain situation or action is not known to have occurred) – nullifying the score of sentiment words that appear in the same fragment. . For example, in the system dictionary it was indicated that the expression не думать (don't think) has a negative sentiment, while this expression did not have this sentiment in one of the analyzed examples: Сам игрок заявил, что пока не думает о переходе в другой клуб (The player said he is not thinking of moving to another club…).

Acknowledgements
Classification features
The bottom line is that if there's a thought word next to the candidate, then it's likely that thought has been expressed about it and could be a product feature. We count the number of documents in which the opinion word ow is in the vicinity of p words within the candidate c.
Experiments 1. Dataset
- Computation of classification features
- Product feature classification
Weirdness-LIB is calculated using the number of documents returned by the "candidate query: market. Another interesting observation is that the PMI calculated using the "same-document" query ("PMI-doc") performs slightly better than the calculated with the question "same sentence" ("PMI-snt").

Conclusion
We strongly believe that such a classic method of text processing as syntactic analysis can significantly improve sentiment analysis performance. Automatic sentiment analysis task faces many problems, such as implicit expression of emotional component in the text, too informal language of reviews and until recently lack of annotated corpus for Russian to measure the quality.
Related works
The main purpose of our participation in ROMIP 2012 was to measure the quality of work of our system and compare it with others to understand if we are on the right track and what we still need to work against.
Method description
- Objects thesaurus
- Syntactic relations used for opinion extraction
- Sentiment dictionary
- Two class classification of blog texts
Then we manually filtered those that were gone, and only 1.5 thousand terms became part of the thesaurus. For example, the phrase "что-то было ухрахным" represents a negative attitude regardless of the object.
Results and further work
Proceedings of the International Conference "Dialog 2012" [Komp'iuternaia Lingvistika i Intellektual'nye Tehnologii: Trudy Mezhdunarodnoj Konferentsii. 2012), Proof of concept statistical sentiment classification at ROMIP 2011, Computational Linguistics and Intellectual Technologies: Proceedings of the International Conference “Dialog 2012” [Komp'iuternaia Lingvistika i Intellektual'nye Tehnologii: Trudy Mezhdunarodsii "Divoalogusi" Konferen, 2012], pp.
Алгоритм сентиментного анализа на основе лингвистических правил
- Морфо-синтаксический анализ
- Сентиментный анализ
- Ключевые слова
- Сентиментные правила
Постановка задачи
- Дорожки РОМИП по сентиментному анализу
- Подготовка системы к сентиментной классификации
- Данные тестирования РОМИП
Результаты системы ATEX и их анализ
Важно отметить, что при сравнительном анализе F-меры и результатов точности система, основанная на правилах, дает высокую F-меру при относительно более низком значении точности.
Выводы и дальнейшая работа
Automatic evaluation of machine translation (MT) quality is about calculating the similarity between a system's output and one or more reference translations for a given source text. Note that lexical-based metrics are unable to capture the syntax or semantic structure of sentences; therefore, they are not directly sensitive to the improvement of machine translation systems on these aspects.
Linguistically-motivated evaluation measures
This approach is based on the assumption that measures at different levels capture different aspects of translation quality. Although linguistically-enriched evaluation measures have shown good properties and higher correlation with human assessments in several MT evaluation campaigns, they are still not widely adopted by developers and researchers when doing actual machine translation evaluations and comparisons.
Intelligent MT output and error analysis
The search module, tSearch, is built on top of Asiya and connected to the Asiya online interface. In contrast, the Asiya interface and the tSearch tool together facilitate the qualitative analysis of the evaluation results, but provide a framework to obtain multiple evaluation metrics and linguistic analysis of the translations.
Quality Estimation
In Proceedings of 11th Conference of the European Chapter of the Association for Computational Linguistics (EACL). In Proceedings of the 11th Conference on Theoretical and Methodological Issues in Machine Translation (TMI).
Данные
Для оценки мы выбрали 947 «чистых» предложений (то есть с правильными границами, без паразитных HTML-тегов и т. д.), из них 759 новостных и 188 — из официальных текстов. Все 947 переводов были использованы для автоматической оценки качества перевода, 330 предложений из 947 были отобраны для ручной оценки (190 новостей и 140 официальных текстов).
Ручная и автоматическая оценка
Получить однозначный общий рейтинг систем на основе частичного рейтинга не всегда легко [Callison-Burch et al., 2012]. Помимо ручной оценки, мы также провели автоматизированную оценку с использованием следующих показателей: BLEU [Papineni et al.
Результаты
Это наблюдение дополнительно подтверждает тот факт, что автоматические метрики систематически недооценивают качество систем МП, основанных на правилах [Béchar et al., 2012]. Эти результаты согласуются с данными о низкой согласованности между экспертами при оценке систем примерно одного уровня [Callison-Burch et al., 2011].
Заключение
Благодарности
Selected issues of semantic analysis 1. Normalization and paraphrasing
- Semantic definitions of NL words and ontology concepts
- Converse terms
- Evaluation of objects
Since inverse terms refer to the same situation, it is sufficient for a semantic language and ontologies to contain only one term of the pair. Then we will show how it is incorporated into our ontology and used for semantic analysis.
Semantic analysis in ETAP
Related work
Dagiti Proceedings ti maika-49 a Tinawen a Miting ti Asosiasion para iti Komputasional a Lingguistika: Dagiti Teknolohia ti Pagsasao ti Tao—v. Dagiti Proceedings ti maika-49 a Tinawen a Miting ti Asosiasion para iti Komputasional a Lingguistika: Dagiti Teknolohia ti Pagsasao ti Tao—v. Leonid.Evdokimov@promt.ru), ti А. Alexander.Molchanov@promt.ru) ООО «ПРМТ», Abagatan nga Aprika, Rusia.
Related Work
User-generated content (UGC) is material on websites, and occasionally other media sources, produced by website users (who are generally amateurs as opposed to professional editors, copywriters, etc.). Reliability and robustness are basic requirements for an automated machine translation system for processing UGC.
Aim and Objectives
Jie Jiang et al., 2012] report on the adaptation of an automated user message translation system in a multilingual social network. An MT system for handling UGC must 1) be deeply customized for this specific type of content and 2) be able to translate large amounts of text in real time.
Statistical and Linguistic Analysis of the Data provided by TripAdvisor
- Initial Data
- Domain-Specific Dictionaries
- Translation Memory
- Target Language Model
- Quality Estimation System
- Deliverables
A language model is a set of n-grams (word sequences of n-length) and their statistical characteristics. We named the language model built on the Russian review corpus the BigTripAdvisor language model.

Translation Quality Evaluation
Our experts performed linguistic analysis of the PROMT baseline system and the PROMT DeepHybrid system output. 3,291 sentences (78% of the test set) of the PROMT DeepHybrid system output contained changes compared to the PROMT baseline system output.
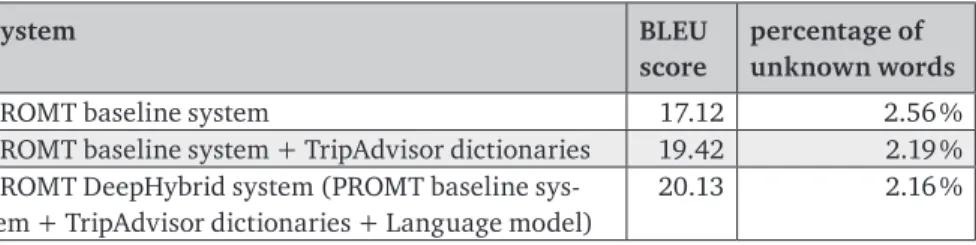
Conclusions
Галинская И. vgoussev@yandex-team.ru), Шматова М. mashashma@yandex-team.ru) Шматова М. mashashma@yandex-team.ru) Яндекс, Москва, Россия. Ключевые слова: статистический машинный перевод, качество машинного перевода, метрики BLEU, опечатки, капитализация, пунктуация. орфографических ошибок на качество машинного перевода СТАТИСТИКА. mescheryakova@yandex-team.ru), Галинская И. vgoussev@yandex-team.ru), Шматова М. mashashma@yandex-team.ru) Шматова М. mashashma@yandex-team.ru) Яндекс, Москва, Россия.
Типичные ошибки в запросах
Опечатки
Отсутствие диакритики
Отсутствие капитализации и/или пунктуации
Наконец, в разделе 5 будут представлены фактические результаты измерений и комментарии к ним. data i miejsce urodzenia adres nr dokumentu urozentijnosti/paszportu → Data i miejsce urodzenia, adres, nr dokumentu urozentija/paszportu 'Дата и место рождения, адрес, номер документа, удостоверяющего личность/паспорта'. при копировании содержимого веб-страницы названия заполняемых полей объединяются в единую последовательность слов);
Характеристика тестовых наборов
Доля запросов с разными типами ошибок в тестовых наборах Язык Диакритика Опечатки
- Методика эксперимента
- Результаты эксперимента
- Приведем результаты подсчетов стандартного BLEU (с учетом пунктуации)
- BLEU без учета пунктуации
- BLEU с учетом капитализации и пунктуации таблица 6
В нашем эксперименте использовались три BLEU-метрики: а) стандартные (рассматривая знаки препинания как отдельные знаки); б) КУПИТЬ без знаков препинания; и в) BLEU, с учетом пунктуации и заглавных букв (т.е. также с учетом разницы между строчными и заглавными буквами в переводе и стандарте). В немецком языке обращает на себя внимание значительно большее влияние на рост BLEU исправления опечаток по сравнению с исправлением ошибок заглавных букв и пунктуации - хотя процент запросов с ошибками второго типа не меньше, даже немного больше, чем первого. (40,2% и 48,8% соответственно, соотношение близко к тому, что мы видели в английской группе).
Заключение
Approach
- Opinion lexicon projection
- Document Sentiment Classification
It has the same number of words as “BL-GT filtered”, but the performance of “ROMIP-GT merged” is higher, so we can say that the quality for sentiment classification is better. Language-independent approach to sentiment analysis (Limsi participation in romip'11) Proceedings of the International Conference Dialog, 2012.

Preface
Statistical Machine Translation — a short overview
Proposed approach
Lexicalized dependency probability (either surface or semantic) is a probability of the dependency link in the parse tree conditioned on lexical classes of parent and child. Lexicalized dependency probability is crucial for determining the correct parse tree and disambiguation of word senses.
As with dependencies, the number of parameters is squared to the number of classes. Thus, we can apply event counting to classes at higher levels of the hierarchy.
Training the probabilistic model
Out of model translation
Evaluation
Philipp Koehn, Hieu Hoang, Alexandra Birch, Chris Callison Burch, Marcello Federico, Nicola Bertoldi, Brooke Cowan, Wade Shen, Christine Moran, Richard Zens, Chris Dyer, Ondrej Bojar, Alexandra Constantin en Evan Herbst.
Abstracts
The main pitfalls of machine learning related to sentiment analysis have been classified and analyzed. APPLICATION OF STATISTICAL METHODS FOR DETECTION OF PROSODIC BOUNDARIES AND PREDICTION OF INTERRUPTION DURATION IN THE RUSSIAN TTS SYSTEM. chistikov@speechpro.com), Speech Technology Center Ltd, St. The article discusses statistical methods for predicting the positions and duration of prosodic breaks in the Russian TTS system. The results and avenues for further investigation and improvement are discussed in the final section. CONTEXT-FREE LEXICON TRANSLATION USING A PARALLEL CORPUS. alexander.ulanov@hp.com), Sapozhnikov G. gsapozhnikov@gmail.com), Hewlett-Packard Labs Russia, St. The paper deals with multilingual sentiment analysis.
Авторский указатель