Assessment of pollutant level and forecasting water pollution of Chennai coastal, TamilNadu using R
Texto
(2) PADMANABAN P N et al.: FOREC CASTING WAT TER POLLUTIO ON USING R. 1421. Fig. 1 — Location of Cheennai costal and sample collecteed spot. multitude of anthropogeenic impacts attributable to accelerated population growth g arounnd 10 millionn15. Developmennt of small and large-scale industriies, expansion of o harbors, tourism t related activities in coastal zonee, disposal of o municipall and industrrial wastes, numerous recrreational annd commerccial activities nott only degrad de the quality of coastal waater but also posee a serious heealth hazard to t marine biootas 16 and human lives . w Around 32 coastal stations in Chennai were identified ass polluted areeas17. The detaails of sampling points are mentioned in i Table 1. Regulation of pollution coontrol board d advises to discharge the drainage/wasste water at a depth of more than 20 metters in the oceann for healthier dispersal and diffusionn18. Discharging at this leevel helps to dilute the concentration of organic and inorgannic contaminaants of wastewateer19. This typee of dischargee always usedd to maintain thhe concentraation of thee organic and a inorganic maaterial below w the thresholdd level whichh is not harmful to the aq quatic and living l beings17. On contraryy to this, in Chennai city most of the organicc and inorg ganic contam minants of the drainage/wasstewater are disposed d in thhe coast itselff17. The coasstal surface waters of north Chennnai, Coovum andd Adyar riveer mouth weere identifiedd to have high concentrations c s of nutrientts, low DO and a high BOD and COD D17. Thereforre, this stuudy concentratedd on the Chen nnai coastal areas a to identtify the level andd forecasting the t water polllution.. p Datta consists off different waater quality parameters of coaastal over thee period of 355 years (1980 to 2015). The yearly y water quality data of physical, chemical and biiological paraameters data were w obtainedd from the Tamillnadu Pollutiion Control Board (TNP PCB).The physiccal, chemiccal and biological b p parameters compuuted for quallity analysis are Temperaature (C), pH, Turbidity T (NT TU), Dissolvved Oxygen (mg L−1), Nitritee (mg L−1), Phosphate P (mgg L−1), Total Dissolved D organic Solidss (TDS), Electrical E C Conductivity, Nitratte(mg L−1), BOD(mg L−1), COD(m mg L−1), Chlorrophyll(mg L−1) and Tottal Coliform Bacteria (TCB) (MPN / 100ml). Pollutants P inn coastal enviroonment derivved from bothh point and non-point sourcees of coastaal water (T Table 2). Asssociating Generral Coastal Cater Quuality standaards, the conceentration and dispersion off the various physical, biologgical and cheemical param meters of coasstal water were evaluated e (Taable 3). Thee figure 2 shows the methodologyy applied evaluating pollution and for concentration forecaasting pollutiion level. An A Automaticc forecast of univariate u tim me series is always required while handling larrge amount of o data 20. This T study practices two automatic a foorecasting algorithms a 2 in thee forecast pacckage of R 21 . The first algorithm in R package is about a automaatic forecastiing based on thhe state sppace modelss called exxponential smootthing methodds and the second algoorithm is forecaasting with AR RIMA modells 22,23..
(3) INDIAN J. MAR. SCI., VOL. 47, NO. 07, JULY 2018. 1422. Table 1 — Sampling station details ID 1 2 3 4 5 6 7 8 9 10 11 12 13 14 15 16 17 18 19 20 21 22 23 24 25 26 27 28 29 30 31 32. Locations Ennore Creek Talakuppam (groundwater) Talakuppam Opposite to Urea Factory Ennore ETPS (groundwater) Annai Sivagami Nagar Bharathiar Nagar Near Amman Temple Bharathiar Nagar (groundwater) Near MRF company Near MRF (groundwater) Near Kanni Koil Ennore Near Kanni Koil (groundwater) Susandherpuram Vannarpet Near Chennai Harbour New CISF Opposite to CISF (North of Cooum) Cuvuum River Mouth Temple Near Cooum river mouth (groundwater) Marina Beach near Anna Samathi Near M.G.R Samathi Marina Opp. Presidency College Marina Opp. to Railway station Opp. to Madras University Hostel (groundwater) Opp. Queen Maris College Opp. Santhome Church Opp. Rajiv Gandhi Nagar Adayar river mouth Near Adayar river Opp Adayar Old Bungalow Near Besant Nagar beach (groundwater) Near Ashtalakshmi Temple Besant Nagar. Latitude 13014'0 51"N 13013'4 44"N 13013'3 23"N 13012'8 10"N 13012'2 03"N 13012'7 28"N 13011'1 20"N 13011'1 52"N 13010'5 40"N 13010'5 41"N 13009'4 09"N 13009'4 41"N 13008'7 37"N 13008'1 24"N 13008'4 19"N 13004'4 29"N 13008'1 16"N 13004'0 12"N 13004'1 46"N 13003'7 41"N 13003'7 50"N 13003'3 58"N 13003'6 58"N 13003'2 49"N 13002'3 11"N 13001'9 24"N 13001'5 13"N 13001'5 23"N 13000'7 60"N 13000'4 34"N 13000'3 20"N 12059'3 11"N. Longitude 80019'9 11"E 80019'7 04"E 80019'7 04"E 80019'5 03"E 80019'1 19"E 80019'1 49"E 80019'0 29"E 80018'9 54"E 80018'7 17"E 80018'7 27"E 80018'3 37"E 80018'3 50"E 80018'0 17"E 80017'8 27"E 80017'5 23"E 80017'5 33"E 80017'9 21"E 80017'9 33"E 80017'9 22"E 80017'9 41"E 80017'9 54"E 80017'9 44"E 80017'9 46"E 80016'9 31"E 80016'9 32"E 80016'9 45"E 80016'9 49"E 80016'9 56"E 80016'9 12"E 80016'9 57"E 80016'9 06"E 80016'9 09"E. Table 2 — Point and non-point sources of pollution in Chennai coastal water S. No 1 2 3 4 5 6. Point / Non-point Sources Municipal sewage wastes Combined sewer overflows Industrial wastes Urban runoff Fly ash Mining/dredging. Pollutants BOD, bacteria, nutrients, ammonia, toxic chemicals BOD, bacteria, nutrients, ammonia, turbidity, total dissolved solids, toxic chemicals Toxic chemicals and BOD Turbidity, bacteria, nutrients, total dissolved solids, toxic chemicals Nutrients, turbidity, toxic chemicals Turbidity, acids, toxic chemicals, total dissolved solids. Exponential smoothing is a technique use to handle the time series spatial data22. Exponential smoothing methods were originally classified by Pegels' taxonomy22. This project deals with the innovations in state space modelling framework and that algorithm implemented by means of the ‘ets( )’ function in the forecast package20. Highly concentrated water quality physical, chemical and biological parameters were identified, analysed and forecasted using automated exponential smoothing. The ‘etsfit’ is of class “ets” holds all information about the fitted model which includes the parameter’s value24. For an instance,. printing the parameter object (etsfit1) shows the main substance of interest, such as smoothing parameters, initial states and model selection24. Autoregressive Integrated Moving Average (ARIMA) models is a simplification of an autoregressive moving average (ARMA) model which is used for forecasting the univariate time series data23. The study covers with an ARIMA model for forecasting the highly concentrated polluted in Chennai coastal. A non-seasonal ARIMA model is classified as an "ARIMA(p,d,q)" model, where ‘p’ is the number of autoregressive conditions, ’d’ is the number of non-seasonal differences and ‘q’ is the number.
(4) PADMANABAN P N et al.: FOREC CASTING WAT TER POLLUTIO ON USING R. of lagged forrecast errors in n the predictionn equation25. The T function ‘autoo-arima ( )’ waas used in this project for fittting the models. The project covers c differeent fitted moddels such as ARIM MA (0, 1, 1),, ARIMA (2,11,2) and ARIM MA (0,1,0)24. Results and d Discussion The various physicall, chemical and biologiical parameters were w plotted with the aidd of time serries. 1423. analyssis. Mean vaalue of each parameter is analysed and plotted in figuure 3 and 4. Graph G shows that each meter time seriies is analyseed with respecct to plots, param whichh has corressponding parrameter’s vaalue from the yeear 1980 to 20015. Red linee assists to diffferentiate the permissible pollutant value v and standard conceentration of parameters. Mean value of each param meter is analyysed and plotted with refference to the geeneral coastaal water quallity standards. Finally,. Table 3 — General G coastal water w quality stanndards of severaal parrameters Parameters Standarrds PH. 7.8–8.3. Temperature (◦C). 30. Turbidity (NTU U). 10 NTU U or less. Total Suspendeed Sediments (TS SS). 25 mg L−1 or less. Dissolved Oxyggen (DO). − 4 mg L−1 or more. − Biochemical Oxygen Demand (BOD) 30mgL−1 or les. Chemical Oxyggen Demand CO OD. 250 mg L−1 or less. Total Coliform Bacteria. 200 MP PN / 100ml or lesss. Nitrate. 10 mg L−1 or less. Nitrite. 10 mg L−1 or less. Total Nitrogen. − 1 mg L−1 or less. P Phosphorus as Phosphate. 0.1 mg L−1 or less. Chlorophyll-a. 15 mg L−1or less. Fig. 2 — Methodoology applied for evaluatingg pollution concenntration and foreccasting pollutionn level. Fig. 3 — Time T series analyysed plot and varriance of Chloroophyll, BOD, CO OD, EC, DO andd Nitrite.
(5) 1424. INDIIAN J. MAR. SC CI., VOL. 47, NO O. 07, JULY 20118. Fig. 4 — Time seriees analysed plot and variance off Nitrate, TCB, PH, P Phosphate, TDS, T Temperaturre and Turbidityy. the output shows sev veral param meters such as chlorophyll, BOD, COD D, nitrite, TCB, phosphaate, temperature and turbiditty falls abovve the threshold level which is detrimenttal to the aquuatic life, living beings and too the environm ment. The estim mated seasonal factors are given for the months Januuary-Decemb ber, which iss the same for each year. Highly con ncentrated paarameters were w plotted to estimate the trend, t seasonnal, and randdom components of the time seeries. The figgure 5 shows the original tim me series, estimated e trrend, estimaated seasonal, andd estimated of o the irregulaar componentt of parameters temperature an nd BOD. In the tem mperature plott, the trend coomponent shoows a peak about 30C in 198 87, 1995 andd 2013.There are many peaks in BOD tren nd componennts and it rangges above 50 mggL−1 in 1990, 1993, 2003, 2007 2 and 2010. The figuure 6 showss the original time seriies, estimated treend, estimated seasonal, and a estimatedd of. p of parameters p the irregular component plot Chlorrophyll and Nitrite. The trend compponent in chloroophyll has peaked p abovee 15 mgL−1 in 1984, 1987, 1997 and 2007. 2 In the nitrite trendd has two peaks about 150 mgL m −1 in 1983 and 2013. Thee figure 7 shows the original tim me series, estimaated trend, esstimated seasonal, and esttimated of the irrregular component plot off parameters Phosphate P and TCB. T Phosphaate trend show ws peak abovve 0.1 mg L−1 inn 1982, 1998 and 2010. Thhe TCB trendd analysis clearlyy shows diffeerent peaks raanges above 200 2 MPN. Thee figure 8 shows the original tim me series, estimaated trend, esstimated seasonal, and esttimated of the irrregular compponent plot off parameters Turbidity and COD. C The trennd analysis of o turbidity haas several peaks above 10 NT TU during 19982, 1994, 19997, 2004 and 2011. The CO OD trend anallysis clearly shows s the param meter value fallls above 2500 mg L−1 in 19982, 1996, 2004 and 2011..
(6) PADMANABAN P N et al.: FOREC CASTING WAT TER POLLUTIO ON USING R. Fig. F 5 — Trend, Seasonal and Raandom componeents of Temperatture and BOD. Fig. F 6 — Trend, Seasonal and Raandom componeent of Chlorophyyll and Nitrite. Fig. 7 — Trend, Seassonal and Randoom component of o Phosphate andd CalifornBacteriia. 1425.
(7) 1426. INDIIAN J. MAR. SC CI., VOL. 47, NO O. 07, JULY 20118. Fig. 8 — Trendd, Seasonal and Random compoonent of Turbiditty and COD. Fig. 9 — Differrent parameter forrecast and 80% & 90 % prediction intervals using exxponential smoothhing state space models m of differentt parameters. Highly concentrated physical, chemical and a biological parameters aree undergone with automaatic exponential smoothing for fo forecastingg the parameeter value. The output o of each h parameter cllearly shows the value falls above the permissible level which is harmful to living beings and aquatiics. Forecasting output show ws the temperature may vary v in-betweeen 27 C and 29 2 C which is i nearby threeshold value.. In same way, the BOD also a lies in non-permissiible threshold vaalue between n 50 mgL−1 and a 100 mgL L−1. −1 Chlorophyll forecasting falls f betweenn 13 mg L and a 25 mg L−1 which w is mucch greater thaan the threshhold value accordding to coasttal quality sttandards. In the same way paarameter valu ue, greater thaan the threshhold in COD foreecasting, it liies between 230 2 mg L−1 and a. 900 mg m L−1. The figure f 9 show ws different parameter p forecaast of 80% & 90 % preddiction intervvals using exponnential smoothhing state spaace models. Figgure 10 shoows differennt parameter forecast and 80% & 900 % predicction intervaals using exponnential smooothing state space moddels. The nitritee forecasting clearly show ws the values are above 10 mg m L−1 whicch is higherr than the threshold value. In phosphhate forecastting the rannges vary betweeen 0.1 mg L−1 and 0.3 mg m L−1 whicch is also abovee the thresholld value of coastal standaards. TCB forecaast to clearlyy shows it liees above 200 MPN. In the same s way, the t turbidityy forecast shhows the ranges above thhe thresholdd value whhich falls betweeen 10 NTU to t 60 NTU..
(8) PADMANABAN P N et al.: FOREC CASTING WAT TER POLLUTIO ON USING R. 1427. Fig. 10 — Diffferent parameter forecast and 80% % & 90 % prediction intervals using u exponentiaal smoothing statte space models. Fig. 11 — Diffferent parameterss Temperature, Chlorophyll, C BO OD and COD foreecast and 80 % and a 95% predicttion intervals obttained using ARIMA modells. Differing from an expo onential smooothing algorithhm, me homoscedaasticity, whichh is the ARIMA models assum not always unsuitable. Therefore, transformatioons are applicabble. The hig ghly concenttrated physiccal, chemical annd biologicall parameters are undergoone with an ARIMA A mo odel for forrecasting vallue.. The output o of eaach parameteer clearly shhows the value lies above the permisssible level which is harmfful to living beings b and aqquatics. The figure 11 and 12 1 shows thee different paarameter forecast and 80 % and 95% prediction p inttervals obtainned using ARIM MA models..
(9) 1428. INDIIAN J. MAR. SC CI., VOL. 47, NO O. 07, JULY 20118. Fig. 12 — Diffferent parameterss Nitrite, TCB, Phosphate P and Turbidity forecastt and 80 % and 95% 9 prediction intervals i obtaineed using ARIMA modells. Conclusionss The studyy identifies high h concentration of BO OD, Chlorophyll,, COD, Nitrite, N Phoosphate, TC CB, Temperaturee and Turbiidity in the coastal watter. Forecasting values also higher than thee threshold lim mit of internatioonal standardss. As a resullt of time serries analysis, wee found that the coastal region is gettting more toxic in the paast recent years y and the concentration of the paraameters will be increasedd to higher level in near futurre say by 20220, thus moddern techniques and a more disp persion modeels are necessary to predict thhe concentration levels annd to reduce the level of polllution in the Coovum C and Adayar estuary unless it will w directly affect the coastal c area of Chennai. Prroper drainag ge network and pre-treaated plant is esssential to dissperse the huuge quantity of waste water into the deep p ocean. Ourr results derivved at, emphassis the autthorities of waste waater managementt and pollutio on control aboout the polluttion spots and priority mitiigation areass from coasstal wetland degrradation in Ch hennai area.. 2. 3. 4. 5 6. 7. 8. References 1. Stow D.A A., Hope A., MccGuire D., Verbbyla D., Gamonn, J., Huemmricch F., Houston S., Racine C., Sturm S M., Tape K., Remote sensing s of vegeetation and lannd-cover changee in. Arrctic Tundra Ecosystems. E Reemote Sens. Ennviron, 89, (22004), 281–308. Laambin E.F., Turnner B.L., Geist H.J., H Agbola S.B B., Angelsen A., Folke C., Bruce B J.W., Coomes C O.T., Dirzo, R., Geeorge, P.S., The T causes of land-use and land-cover chhange: Moving beyond b the mytths. Glob. Envirron. Chang, 111, (2001), 261–269. Viisalatchi A., Paadmanaban R, Land L Use and Land L Cover M Mapping and Shhore Line Channges Studies inn Tuticorin Cooastal Area Usinng Remote Senssing. Int. J. Rem mote Sens. 1, (22012), 1–12. M Monishiya B.G., Padmanaban R, Mapping and a change deetection analysis of marine reesources in Tuuticorin and Veembar group off Islands using remote r sensing. Int. J. Adv. Foor. Sci. Manag, 1, (2012), 1, 1–116. Sqquires G.D, Urrban Sprawl Causes, C Consequuences and Poolicy Responsess; The Urban Innstitute Press: Washington, W DC C, USA, (2002).. Caabral P, Feger C, Levrel H, Chambolle M, Basque D, Asssessing the im mpact of land-coover changes onn ecosystem seervices: A firsst step towardd integrative planning p in Boordeaux, France. Ecosyst. Serv, 22, 2 (2016), 318––327. Caabral P, Santo J.A, J Augusto G,, Monitoring Urrban Sprawl annd the Nationaal Ecological Reserve R in Sinntra-Cascais, Poortugal: Multipple OLS Linear Regression Model Evvaluation, J. Urbban Plan. Dev, 137, 1 (2011), 346––353. Jaayaprakash M, Senthil Kumar R,, Giridharan L, Sujitha, S S.B, Saarkar S.K, Jonathhan M.P, Bioacccumulation of metals m in fish sppecies from waater and sedimeents in macrotiidal Ennore creek, Chennai, SE E coast of India: A metropolitann city effect, Eccotoxicol. Enviroon. Saf, 120, (20015), 243–255..
(10) PADMANABAN et al.: FORECASTING WATER POLLUTION USING R 9. 10 11 12. 13. 14. 15. 16. Gowri V.S, Ramachandran S, Ramesh R, Pramiladevi I.R, Krishnaveni K, Application of GIS in the study of mass transport of pollutants by Adyar and Cooum Rivers in Chennai, Tamilnadu. Environ. Monit. Assess, 138, (2008), 41–49. Aithal B.H, Ramachandra T.V, Visualization of Urban Growth Pattern in Chennai Using Geoinformatics and Spatial Metrics, J. Indian Soc. Remote Sens, 44, (2016), 617–633. Jat M.K, Garg P.K, Khare D, Monitoring and modelling of urban sprawl using remote sensing and GIS techniques. Int. J. Appl. Earth Obs. Geoinform, 10, (2008), 26–43. Sudhira H.S, Ramachandra T.V, Jagadish K.S, Urban sprawl pattern recognition and modelling using GIS. In Proceedings of the 2003 Map India, New Delhi, India, 1–9, (2003), 28–30. Moghadam H.S, Helbich M, Spatiotemporal urbanization processes in the megacity of Mumbai, India: A Markov chains-cellular automata urban growth model, Appl. Geogr, 40, (2013), 140–149. Dewan A.M, Yamaguchi Y, Land use and land cover change in Greater Dhaka, Bangladesh: Using remote sensing to promote sustainable urbanization, Appl. Geogr, 29, (2009), 390–401. Dewan A.M, Yamaguchi Y, Rahman M.Z, Dynamics of land use/cover changes and the analysis of landscape fragmentation in Dhaka Metropolitan, Bangladesh. GeoJournal, 77, (2012), 315–330. Poelmans L, Van Rompaey A, Computers, Environment and Urban Systems Complexity and performance of urban. 17 18. 19 20. 21 22 23 24. 1429. expansion models. Comput. Environ. Urban Syst, 34, (2010),17–27. Padmanaban R, Integrating of Urban Growth Modelling and Utility Management System using Spatio Temporal Data Mining. Int. J. Adv. Earth Sci. Eng, 1, (2012),13–15. Padmanaban R, Modelling the Transformation of Land use and Monitoring and Mapping of Environmental Impact with the help of Remote Sensing and GIS. Int. J. Adv. Altern. Energy Environ. Ecol, 1, (2012), 36–38. Herold M, Goldstein N.C, Clarke K.C, The spatiotemporal form of urban growth: Measurement, analysis and modeling. Remote Sens. Environ, 86, (2003), 286–302. De Oliveira Filho, F.J.B, Metzger J.P, Thresholds in landscape structure for three common deforestation patterns in the Brazilian Amazon. Landsc. Ecol, 21, (2006), 1061–1073. Tv R Aithal, B.H Sanna D.D, Insights to urban dynamics through landscape spatial pattern analysis. Int. J. Appl. Euarth Obs. Geoinform, 18, (2012),329–343. Byomkesh T, Nakagoshi N, Dewan A.M, Urbanization and green space dynamics in Greater Dhaka, Bangladesh. Landsc. Ecol. Eng, 8, (2012), 45–58. Ferdinent J, Padmanaban R, Development of a Methodology to Estimate Biomass from Tree Height Using Airborne Digital Image. Int. J. Adv. Remote Sens. GIS, 2, (2013), 49–58. Shanmugam P, Neelamani S, Ahn Y.H, Philip L, Hong G.H, Assessment of the levels of coastal marine pollution of Chennai city, Southern India. Water Resource, Manag, 21, (2007), 1187–120..
(11)
Imagem

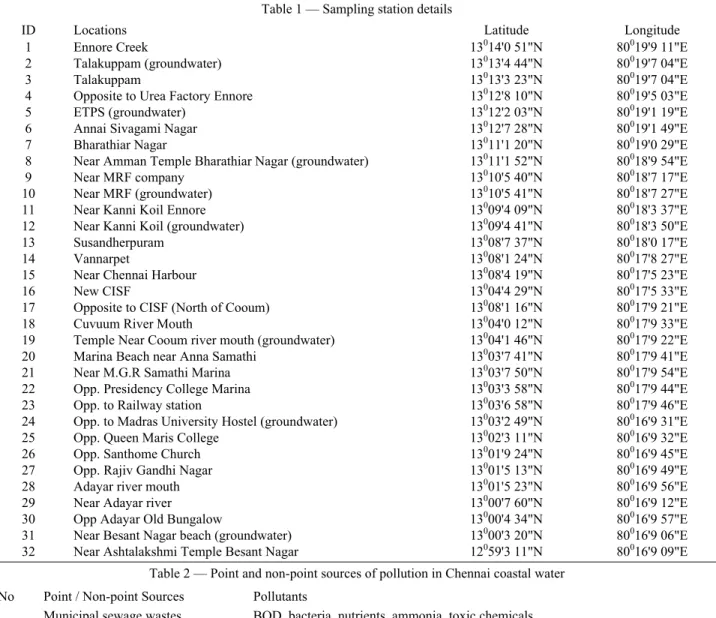
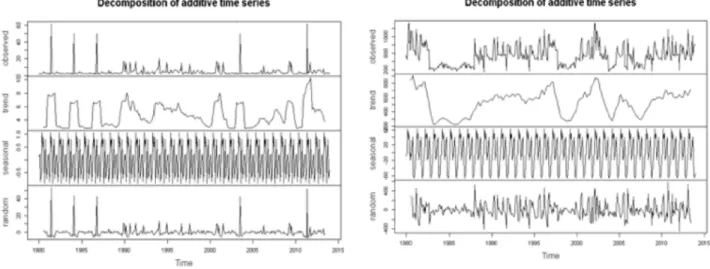
Documentos relacionados
Thus, the main purpose of this study was to determine the influence of diversity within the board on decisions affecting companies’ capital structure, and the basic hypothesis tested
Assessment of biotic and water quality indices in Cheshmekile Tonkabon River using benthic invertebrates communities and the physical and chemical parameters of water.. Effects
soil map and climate data) to predict the water quantity and quality of the ungauged basin using the MapWindow version of SWAT (MWSWAT),.. • Using the Data Uncertainty Engine (DUE)
The probability of attending school four our group of interest in this region increased by 6.5 percentage points after the expansion of the Bolsa Família program in 2007 and
I could propose that all of these, perhaps with the exception of the last one, relate to preserving or enhancing the quality of life, or at least that aspect of it that relates
Ousasse apontar algumas hipóteses para a solução desse problema público a partir do exposto dos autores usados como base para fundamentação teórica, da análise dos dados
social assistance. The protection of jobs within some enterprises, cooperatives, forms of economical associations, constitute an efficient social policy, totally different from
Esse artigo visa apresentar determinado produto e elaborar uma análise de mercado para comercialização do Gelato Italiano Artesanal, onde a principal responsável