Design of Fuzzy Neural Network for Function Approximation and Classification
Texto
Imagem
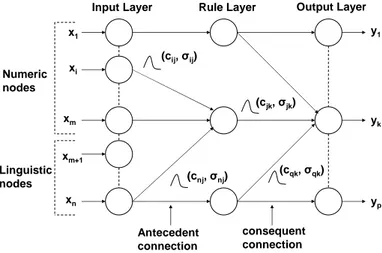
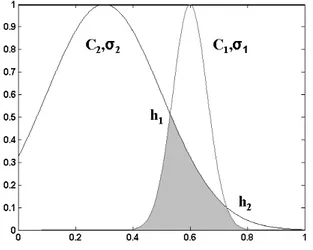
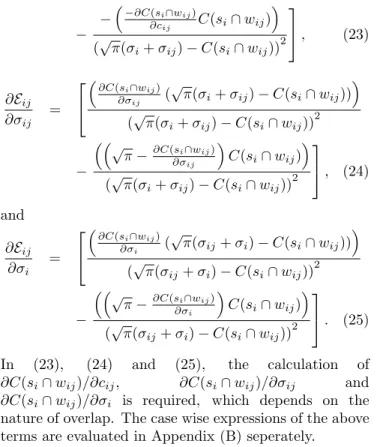

Documentos relacionados
A mulit-layer artificial neural network, with a backpropagation training algorithm and 11 neurons in the input layer, was used.. In this type of network, network inputs are
The application of neural network allowed us to establish a new form of project evaluation based on perceptron learning network and classification of quality standards for services
The number of neurons in input layer, hidden layer and output layer of this neural network were kept as 6, 11 and 1, respectively (Fig. This ANN was first trained with
For the design of the Dumb and Deaf Special Interface (DDSI) Expert Systems, the neural network model is implemented.. Architecture of
The model consists of two distinct parts, a traditional neural network consisting of input layer, number of hidden layers and an output layer with biasing neuron,
In this paper, a robust 4-layer Convolutional Neural Network (CNN) architecture is proposed for the face recognition problem, with a solution that is capable of handling
Method: A neuro-fuzzy system was developed using the NEFCLASS (NEuro Fuzzy CLASSIfication) architecture and an artificial neural network with backpropagation learning
Three feed-forward neural network models characterized by a similar structure (five neurons in the input layer, one hidden layer and one neuron in the output layer) are