Faults Classification of a Scooter Engine Platform Using Wavelet Transform and Artificial Neural Network
Texto
Imagem
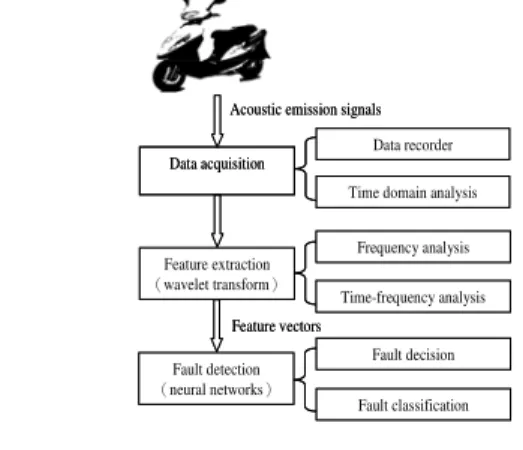
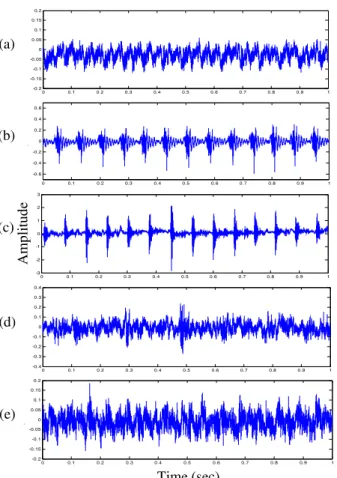


Documentos relacionados
tabLe 2 - Result of discriminant analysis, according to the percentage of correct classification of the heterotic groups proposed by the artificial neural network technique for
Method: A neuro-fuzzy system was developed using the NEFCLASS (NEuro Fuzzy CLASSIfication) architecture and an artificial neural network with backpropagation learning
[r]
In this paper a new approach for block feature based image fusion is implemented using multi wavelet transform. and neural networks and a qualitative analysis has been done for
Abstract: This paper presents an efficient wavelet and neural network (WNN) based algorithm for distinguishing magnetizing inrush currents from internal fault
Automated Breast Cancer Diagnosis based on GVF-Snake Segmentation, Wavelet Features Extraction and Neural Network Classification.. Abderrahim Sebri, Jihène Malek,
In line with this, artificial neural network technology of backpropagation neural network (BPNN), radial basis function neural network (RBFNN) and generalized regression
The comparison of classification in artificial and fuzzy neural networks technique has been conducted to separate classes of forest from non-forest using SPOT satellite