Prediction of ferric iron precipitation in bioleaching process using partial least squares and artificial neural network
Texto
Imagem

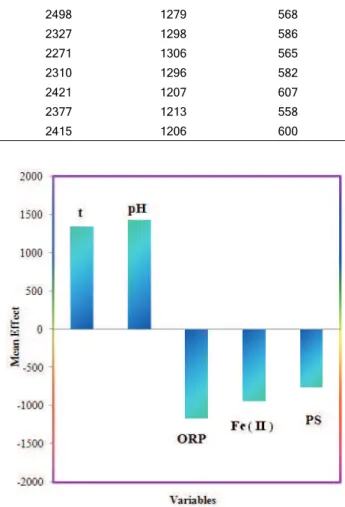
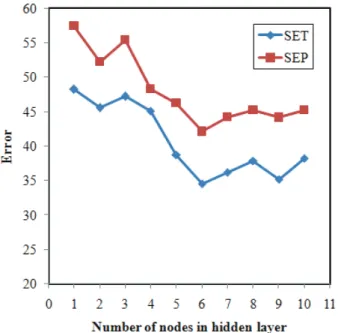

Documentos relacionados
Comparison between principal component, partial least squares and back propagation neural network analyses for accuracy of measurement of selected soil properties with visible and
Method: A neuro-fuzzy system was developed using the NEFCLASS (NEuro Fuzzy CLASSIfication) architecture and an artificial neural network with backpropagation learning
This study compared two prediction models, an Artificial Immune System (AIS) and Artificial Neural Network (ANN) for predicting the future index value, trend
The objective of this study is to investigate the possibility for the prediction of abrasiveness characteristics of thermal coal abrasive index using neural
In this study, a predictive controller using a feed- forward neural network with online adaptation of the parameters was used in the temperature control of a batch process for
In this paper, genetic algorithm based artificial neural network hybrid prediction model is proposed to foretell surface roughness and tool wear.. A multiple
The preparation of SPION was carried out by using two different methods, using an iron-oleate complex (Mag-1 sample) or the co-precipitation of ferrous and ferric chloride
In line with this, artificial neural network technology of backpropagation neural network (BPNN), radial basis function neural network (RBFNN) and generalized regression