Essays on mixed-frequency data : forecasting and unit root testing
Texto
(2)
(3) Universidade de Lisboa ISEG - Instituto Superior de Economia e Gest˜ao Page 1 of 1. Essays on Mixed-Frequency Data: Forecasting and Unit Root Testing ´ udia Filipa Pires Duarte Cla http://aquila1.iseg.ulisboa.pt/aquila/getFile.do?method=getFile&fileId=555679&_req... 28-05-2015. Orientador(es): Prof. Doutor Paulo Manuel Marques Rodrigues Prof. Doutor Jo˜ao Carlos Henriques da Costa Nicolau Tese especialmente elaborada para obten¸c˜ao do grau de Doutor em Economia J´ uri: Presidente: Prof. Doutor Manuel Fernando C´ılia de Mira Godinho, Professor Catedr´atico e Presidente do Conselho Cient´ıfico do Instituto Superior de Economia e Gest˜ao da Universidade de Lisboa Vogais: Doutor Antonio Manuel Pedro Afonso, Professor Catedr´atico do lnstituto Superior de Economia e Gest˜ao da Universidade de Lisboa Doutor Lu´ıs Miguel Rainho Catela Nunes, Professor Associado da Faculdade de Economia da Universidade Nova de Lisboa Doutor Paulo Manuel Marques Rodrigues (orientador) Professor Associado Convidado da Faculdade de Economia da Universidade Nova de Lisboa Doutor Lu´ıs Filipe Farias de Sousa Martins (relator) Professor Auxiliar com Agrega¸ca˜o do ISCTE -lnstituto Universit´ario de Lisboa Doutor Nuno Ricardo Martins Sobreira (relator) Professor Auxiliar Convidado do lnstituto Superior de Economia e Gest˜ao da Universidade de Lisboa 2016.
(4)
(5) iii. To my parents.
(6)
(7) Contents Resumo. xi. Abstract. xiii. Acknowledgments. xv. Introduction. xvi. 1 Macroeconomic Forecasting with MIDAS 1.1 Introduction . . . . . . . . . . . . . . . . . . . . . . . . . . 1.2 Overview of the literature . . . . . . . . . . . . . . . . . . 1.3 MIDAS modelling . . . . . . . . . . . . . . . . . . . . . . . 1.3.1 Background . . . . . . . . . . . . . . . . . . . . . . 1.3.2 From the approximate mixed-frequency regression DAS regressions . . . . . . . . . . . . . . . . . . . . 1.3.3 Autoregressive augmentation of MIDAS regressions 1.4 Design of the nowcasting and forecasting exercise . . . . . 1.5 Empirical results . . . . . . . . . . . . . . . . . . . . . . . 1.5.1 Single-variable models . . . . . . . . . . . . . . . . 1.5.2 Pooling forecasts . . . . . . . . . . . . . . . . . . . 1.5.3 Multi-variable models . . . . . . . . . . . . . . . . . 1.6 Conclusion . . . . . . . . . . . . . . . . . . . . . . . . . . . Acknowledgments . . . . . . . . . . . . . . . . . . . . . . . . . . Bibliography . . . . . . . . . . . . . . . . . . . . . . . . . . . . Appendices . . . . . . . . . . . . . . . . . . . . . . . . . . . . .. . . . .. 1 1 6 10 11. . . . . . . . . . . .. 14 20 28 32 32 39 42 44 45 46 54. 2 Covariate-augmented unit root tests with mixed-frequency data 2.1 Introduction . . . . . . . . . . . . . . . . . . . . . . . . . . . . . . . 2.2 Covariate-augmented unit root tests . . . . . . . . . . . . . . . . . . 2.2.1 The CADF test . . . . . . . . . . . . . . . . . . . . . . . . . 2.2.2 Tests with GLS demeaning . . . . . . . . . . . . . . . . . . .. 61 61 63 66 70. v. . . . . . . . . . . . . . . . . to MI. . . . . . . . . . . . . . . . . . . . . . . . . . . . . . . . . . . . . . . . . . . ..
(8) 2.3. Mixed-frequency covariate-augmented unit root tests 2.3.1 The MIDAS approach . . . . . . . . . . . . . 2.3.2 The M-CADF test . . . . . . . . . . . . . . . 2.3.3 The M-CADF-GLS test . . . . . . . . . . . . 2.4 Monte Carlo simulation . . . . . . . . . . . . . . . . . 2.4.1 Baseline results . . . . . . . . . . . . . . . . . 2.4.2 The case of a larger sample size . . . . . . . . 2.4.3 Different lags . . . . . . . . . . . . . . . . . . 2.4.4 Different time frequencies . . . . . . . . . . . 2.5 An application to the US unemployment rate . . . . 2.6 Conclusion . . . . . . . . . . . . . . . . . . . . . . . . Acknowledgments . . . . . . . . . . . . . . . . . . . . . . . Bibliography . . . . . . . . . . . . . . . . . . . . . . . . . Appendices . . . . . . . . . . . . . . . . . . . . . . . . . . 3 Unit root tests using mixed-frequency VAR models 3.1 Introduction . . . . . . . . . . . . . . . . . . . . . . . 3.2 Feasible point optimal unit root tests . . . . . . . . . 3.2.1 The ERS test . . . . . . . . . . . . . . . . . . 3.2.2 EJ test . . . . . . . . . . . . . . . . . . . . . . 3.3 Mixed-frequency EJ unit root test . . . . . . . . . . . 3.3.1 VAR-MIDAS approach . . . . . . . . . . . . . 3.3.2 M-EJ test . . . . . . . . . . . . . . . . . . . . 3.4 Monte Carlo simulation . . . . . . . . . . . . . . . . . 3.4.1 Baseline results . . . . . . . . . . . . . . . . . 3.4.2 The case of a larger sample size . . . . . . . . 3.4.3 Different lags . . . . . . . . . . . . . . . . . . 3.5 An application to the US unemployment rate . . . . 3.6 Conclusion . . . . . . . . . . . . . . . . . . . . . . . . Acknowledgments . . . . . . . . . . . . . . . . . . . . . . . Bibliography . . . . . . . . . . . . . . . . . . . . . . . . . Appendices . . . . . . . . . . . . . . . . . . . . . . . . . . Conclusion. . . . . . . . . . . . . . .. . . . . . . . . . . . . . . . .. . . . . . . . . . . . . . .. . . . . . . . . . . . . . . . .. . . . . . . . . . . . . . .. . . . . . . . . . . . . . . . .. . . . . . . . . . . . . . .. . . . . . . . . . . . . . . . .. . . . . . . . . . . . . . .. . . . . . . . . . . . . . . . .. . . . . . . . . . . . . . .. . . . . . . . . . . . . . . . .. . . . . . . . . . . . . . .. . . . . . . . . . . . . . .. 74 76 78 78 79 84 91 93 94 96 99 100 101 106. . . . . . . . . . . . . . . . .. 109 . 109 . 111 . 111 . 114 . 118 . 118 . 121 . 122 . 126 . 134 . 136 . 137 . 141 . 141 . 142 . 147 150.
(9) List of Tables A.1 Single-variable models: relative performance, in terms of RMSFE, against an AR benchmark, for forecast horizon h . . . . . . . . . . A.2 Single-variable models: relative performance, in terms of RMSFE, against a traditional low-frequency quarterly benchmark, for forecast horizon h . . . . . . . . . . . . . . . . . . . . . . . . . . . . . A.3 Single-variable model for different time frequencies, against an autoregressive benchmark, for forecast horizon h . . . . . . . . . . . A.4 Pooled forecasts: relative performance, in terms of RMSFE, against an AR benchmark, for forecast horizon h . . . . . . . . . . . . . . A.5 Multi-variable models, against an AR benchmark, for forecast horizon h . . . . . . . . . . . . . . . . . . . . . . . . . . . . . . . . . .. . 56. . 57 . 58 . 59 . 60. 2.4.1 Simulation design and median of the lag order selected by MAIC (Perron and Qu, 2007) . . . . . . . . . . . . . . . . . . . . . . . . . 83 2.4.2 Finite sample size for unit root tests considering nominal size of 5 per cent, T = 100 . . . . . . . . . . . . . . . . . . . . . . . . . . . . 85 2.4.3 Size-adjusted power of unit root tests, Constant only, T = 100 . . . 88 2.4.4 Size-adjusted power of unit root tests, Time trend included, T = 100 89 2.4.5 Estimated R2 and δ parameters and critical values, Constant only . 90 2.5.1 Unit root tests for US monthly unemployment rate, using jobless claims as covariate . . . . . . . . . . . . . . . . . . . . . . . . . . . 98 2.5.2 Unit root tests for US monthly unemployment rate, using jobless claims as covariate . . . . . . . . . . . . . . . . . . . . . . . . . . . 99 B.1 Asymptotic critical values for CADF t-statistics . . . . . . . . . . . 108 B.2 Asymptotic critical values for the CADF-GLS test . . . . . . . . . . 108 3.4.1 Simulation design and median of the lag order selected by AIC . . . 125 3.4.2 Finite sample size for unit root tests considering nominal size of 5 per cent, T = 100 . . . . . . . . . . . . . . . . . . . . . . . . . . . . 127 3.4.3 Size-adjusted power of unit root tests, Constant only, T = 100 . . . 130 3.4.4 Size-adjusted power of unit root tests, Time trend included, T = 100 131 vii.
(10) 3.4.5 Estimated R2 and critical values of EJ-family unit root tests, Constant only . . . . . . . . . . . . . . . . . . . . . . . . . . . . . . . . 133 3.5.1 Unit root tests for US monthly unemployment rate, using jobless claims as covariate . . . . . . . . . . . . . . . . . . . . . . . . . . . 139 C.1 Asymptotic critical values for the EJ test . . . . . . . . . . . . . . . 149.
(11) List of Figures (3). 1.1. Simulated sequences of the coefficients associated with xt and its lags . . . . . . . . . . . . . . . . . . . . . . . . . . . . . . . . . . . . 1.2 Forecast performance of MIDAS single-variable regressions compared to an AR benchmark, relative RMSFE . . . . . . . . . . . . . 1.3 Forecast performance of MIDAS single-variable regressions compared to a quarterly benchmark, relative RMSFE . . . . . . . . . . 1.4 Best performing single-variable regression, for each forecast horizon, relative RMSFE compared to AR benchmark . . . . . . . . . . . . . 1.5 Single-variable model - Dow Jones Euro Stoxx index - for each forecast horizon, relative RMSFE compared to AR benchmark . . . . . 1.6 Relative RMSFE of MIDAS regressions compared to an AR benchmark . . . . . . . . . . . . . . . . . . . . . . . . . . . . . . . . . . . 1.7 Relative RMSFE of MIDAS regressions compared to a quarterly benchmark . . . . . . . . . . . . . . . . . . . . . . . . . . . . . . . . 1.8 Pooled forecasts - best performing MIDAS models against an AR benchmark for each forecast horizon, relative RMSFE . . . . . . . . 1.9 MIDAS regressions - single-variable models vs. pooled forecasts against an AR benchmark, for each forecast horizon, relative RMSFE 1.10 MIDAS models: pooled forecasts vs. multi-variable models - for each forecast horizon, relative RMSFE against an AR benchmark . 2.1 2.2. 2.3 2.4. Finite sample size distortions vis-`a-vis a nominal size of 5 per cent, T = 100, Constant only . . . . . . . . . . . . . . . . . . . . . . . . . Differences in size-adjusted power between mixed- and low-frequency tests with GLS demeaning for c = −5, −10 and −15, Constant only, T = 100 . . . . . . . . . . . . . . . . . . . . . . . . . . . . . . . . . Finite sample size distortions vis-`a-vis a nominal size of 5 per cent, T = 500 . . . . . . . . . . . . . . . . . . . . . . . . . . . . . . . . . Differences in size-adjusted power between mixed- and low-frequency tests with GLS demeaning for c = −5, −10 and −15, Constant only, T = 500 . . . . . . . . . . . . . . . . . . . . . . . . . . . . . . . . . ix. 22 33 33 34 36 37 37 41 42 43 86. 87 92. 93.
(12) 2.5. 2.6 2.7 3.1 3.2 3.3 3.4 3.5. 3.6. Differences in size-adjusted power between mixed- and low-frequency tests with GLS demeaning for c = −5 by number of lags included in the test regression, Constant only . . . . . . . . . . . . . . . . . 94 Size-adjusted power of mixed- and low-frequency tests for different m, with GLS demeaning, aggregate α = 0.99, Constant only, T = 500 95 Data on US unemployment . . . . . . . . . . . . . . . . . . . . . . . 97 Finite sample size, T = 100, Constant only . . . . . . . . . . . . . . Differences in size-adjusted power between mixed- and low-frequency EJ tests for α = 0.95, 0.90 and 0.85, Constant only, T = 100 . . . . Finite sample size, T = 500, Constant only . . . . . . . . . . . . . . Differences in size-adjusted power between mixed- and low-frequency EJ tests for α = 0.99, 0.98 and 0.97, Constant only, T = 500 . . . . Differences in size-adjusted power between mixed- and low-frequency tests with GLS demeaning by number of lags included in the test regression, Constant only . . . . . . . . . . . . . . . . . . . . . . . . Data on US unemployment . . . . . . . . . . . . . . . . . . . . . . .. 128 132 135 135. 137 138.
(13) Resumo Nas u ´ltimas d´ecadas, os investigadores tˆem tido acesso a bases de dados cada vez mais abrangentes, que incluem s´eries com frequˆencias temporais mais elevadas e que s˜ao divulgadas mais atempadamente. Em contraste, algumas vari´aveis, nomeadamente alguns dos principais indicadores macroecon´omicos, s˜ao divulgados com um desfasamento temporal significativo e com baixa frequˆencia. Esta situa¸c˜ao levanta quest˜oes sobre como lidar com s´eries com frequˆencias temporais diferentes, mistas. Ao longo do tempo, v´arias t´ecnicas tˆem sido propostas. Esta tese debru¸ca-se sobre uma t´ecnica em particular — a abordagem MI(xed) DA(ta) S(ampling), proposta por Ghysels et al. (2004). No Cap´ıtulo 1 eu utilizo a t´ecnica MIDAS para prever o crescimento do PIB na a´rea do euro com base num pequeno conjunto de indicadores, cobrindo s´eries com diferentes frequˆencias temporais e divulgadas com diferentes desfasamentos. Eu comparo o desempenho de um conjunto alargado de regress˜oes MIDAS, utilizando a raiz quadrada do erro quadr´atico m´edio de previs˜ao e tomando como ponto de referˆencia quer regress˜oes autoregressivas, quer multivariadas (bridge models). A quest˜ao sobre a forma de introduzir termos autoregressivos nas equa¸co˜es MIDAS ´e dirimida. S˜ao consideradas diferentes combina¸co˜es de vari´aveis, obtidas atrav´es da agrega¸ca˜o de previs˜oes ou de regress˜oes multivariadas, assim como diferentes frequˆencias temporais. Os resultados sugerem que, em geral, a utiliza¸c˜ao de regress˜oes MIDAS contribui para o aumento da precis˜ao das previs˜oes. Adicionalmente, nesta tese s˜ao propostos novos testes de ra´ızes unit´arias que exploram informa¸c˜ao com frequˆencias mistas. Tipicamente, os testes de ra´ızes unit´arias tˆem baixa potˆencia, especialmente em amostras pequenas. Uma forma de combater esta dificuldade consiste em recorrer a testes que exploram informa¸ca˜o. xi.
(14) adicional de um regressor estacion´ario inclu´ıdo na regress˜ao de teste. Eu avalio se ´e poss´ıvel melhorar o desempenho de alguns testes deste tipo ao explorar dados com frequˆencias temporais mistas, atrav´es de regress˜oes MIDAS. No Cap´ıtulo 2 eu proponho uma nova classe de testes da fam´ılia Dickey-Fuller (DF) com regressores adicionais de frequˆencia temporal mista, tomando por base os testes DF com regressores adicionais (CADF) propostos por Hansen (1995) e uma vers˜ao modificada proposta por Pesavento (2006), semelhante ao filtro GLS aplicado ao teste ADF univariado em Elliott et al. (1996). Em alternativa aos testes da fam´ılia DF, Elliott and Jansson (2003) prop˜oem um teste de ra´ızes unit´arias vi´avel que ret´em propriedades ´optimas mesmo na presen¸ca de vari´aveis determin´ısticas (EJ), tomando por base a vers˜ao univariada proposta por Elliott et al. (1996). No Cap´ıtulo 3 eu alargo o aˆmbito de aplica¸ca˜o destes testes de forma a incluir dados com frequˆencia temporal mista. Dado que para implementar o teste EJ ´e necess´ario estimar modelos VAR, eu proponho um modelo VAR-MIDAS n˜ao restrito, parcimonioso, que inclui s´eries de frequˆencia temporal mista e ´e estimado com t´ecnicas econom´etricas tradicionais. Os resultados de um exerc´ıcio de Monte Carlo indicam que os testes com dados de frequˆencia temporal mista tˆem um desempenho em termos de potˆencia melhor do que os testes que agregam todas as vari´aveis para a mesma frequˆencia temporal (necessariamente a frequˆencia mais baixa). Os ganhos s˜ao robusto a` dimens˜ao da amostra, `a escolha do n´ umero de desfasamentos a incluir nas regress˜oes de teste e a`s frequˆencias temporais concretas. Adicionalmente, os testes da fam´ılia EJ tendem a ter um melhor desempenho do que os testes da fam´ılia CADF, independentemente das frequˆencias temporais consideradas. Para ilustrar empiricamente a utiliza¸ca˜o destes testes, analisa-se a s´erie da taxa de desemprego nos EUA.. Palavras-Chave: Regress˜oes MIDAS, Dados de frequˆencia alta, Previs˜ao, Precis˜ao das previs˜oes, PIB da ´area do euro, Ra´ızes unit´arias, Testes de hip´oteses, Dados de frequˆencia mista, VAR-MIDAS n˜ao restrito, Taxa de desemprego dos EUA..
(15) Abstract Over the last decades, researchers have had access to more comprehensive datasets, which are released on a more frequent and timely basis. Nevertheless, some variables, namely some key macroeconomic indicators, are released with a significant time delay and at low frequencies. This situation raises the question on how to deal with series released at different, mixed time frequencies. Over the years and for different purposes, several techniques have been put forward. This essay focuses on a particular technique — the MI(xed) DA(ta) S(ampling) framework, proposed by Ghysels et al. (2004). In Chapter 1 I use MIDAS for forecasting euro area GDP growth using a small set of selected indicators in an environment with different sampling frequencies and asynchronous releases of information. I run a horse race between a wide set of MIDAS regressions and evaluate their performance, in terms of root mean squared forecast error, against AR and quarterly bridge models. The issue on how to include autoregressive terms in MIDAS regressions is disentangled. Different combinations of variables, through forecast pooling and multi-variable regressions, and different time frequencies are also considered. The results obtained suggest that, in general, using MIDAS regressions contributes to increase forecast accuracy. In addition, I propose new unit root tests that exploit mixed-frequency information. Unit root tests typically suffer from low power in small samples. To overcome this shortcoming, tests exploiting information from stationary covariates have been proposed. I assess whether it is possible to improve the power performance of some of these tests by exploiting mixed-frequency data, through the MIDAS approach. In Chapter 2 I put forward a new class of mixed-frequency covariate-augmented Dickey-Fuller (DF) tests, extending the covariate-augmented. xiii.
(16) DF test (CADF test) proposed by Hansen (1995) and its modified version, similar to the GLS generalisation of the univariate ADF test in Elliott et al. (1996), proposed by Pesavento (2006). Alternatively to the CADF tests, Elliott and Jansson (2003) proposed a feasible point optimal unit root test in the presence of deterministic components (EJ test, hereafter), which extended the univariate results in Elliott et al. (1996). In Chapter 3 I go one step further and include mixed-frequency data in the EJ testing framework. Given that implementing the EJ test requires estimating VAR models, in order to plug in mixed-frequency data in the test regression I propose an unconstrained, though parsimonious, stacked skip-sampled reduced-form VAR-MIDAS model, which is estimated using standard econometric techniques. The results from a Monte Carlo exercise indicate that mixed-frequency tests have better power performance than low-frequency tests. The gains are robust to the size of the sample, to the lag specification of the test regressions and to different combinations of time frequencies. Moreover, the EJ-family of tests tends to have a better power performance than the CADF-family of tests, either with low or mixed-frequency data. An empirical illustration using the US unemployment rate is presented.. Keywords: MIDAS regressions, High-frequency data, Forecasting, Forecast accuracy, Euro Area GDP, Unit root, Hypothesis testing, Mixed-frequency data, Unrestricted VAR-MIDAS, US unemployment rate..
(17) Acknowledgments First of all, I would like to thank my advisers, Professors Jo˜ao Nicolau and Paulo Rodrigues, for guiding me through this unique journey. I would also like to thank Banco de Portugal for giving me the opportunity of pursuing this project, while at work, and for the financial support. As I learned over time, combining regular work with such a long-haul project is not always an easy task. A kind acknowledgement is also dedicated to Ricardo F´elix for his commitment to providing research time amid the regular activities. This project benefited from thoughtful discussions with Paulo J´ ulio, Jos´e Francisco Maria and Christian Schumacher, and from comments of participants at the 24th (EC)2 Conference “The Econometrics Analysis of Mixed Frequency Data”, held at the University of Cyprus, and at “Exchange” (Banco de Portugal seminars). Detailed acknowledgements follow at the end of each chapter. A special thanks is owed to F´atima Teodoro for software assistance. I would like to express my deepest gratitude to Carlos Robalo Marques, whose comments and suggestions were crucial for the development of this project. I thank my colleagues and friends, for their encouragement, friendship and for supporting me throughout this process. A special word is dedicated to Jo˜ao Cardoso, for his generosity, encouragement and care. Finally, I thank my parents for all their love, support and encouragement. For always being there. Cl´audia Duarte Lisbon, July 2015. xv.
(18) Introduction In the last decades, researchers have had access to more and more comprehensive datasets. Not only the number of variables available has increased significantly, but data are also released on a more frequent and timely basis. Nevertheless, some variables, namely some key macroeconomic indicators, are released with a significant time delay and at low frequencies. This situation raises the question on how to deal with this diversity of data, in particular on how to deal with series released at different, mixed time frequencies. Over the years and for different purposes, several techniques have been put forward in order to combine data with different frequencies and exploit timely releases of high frequency data. This thesis focuses on one particular technique — the MI(xed) DA(ta) S(ampling) framework, proposed by Ghysels et al. (2004). In Chapter 1 I use MIDAS for forecasting in an environment with different sampling frequencies and asynchronous releases of information. The goal is forecasting quarterly euro area GDP growth using a small set of selected indicators. I run a horse race between a wide set of MIDAS regressions - original and multiplicative, with and without autoregressive terms, with and without current period information - and evaluate their performance, in terms of root mean squared forecast error, against AR and quarterly bridge models. Different combinations of variables, through forecast pooling and multi-variable regressions, and different time frequencies, namely daily, weekly and monthly frequencies, are also considered. Adding to the extensive comparison between MIDAS models, I contribute to the existing literature by disentangling the question on how to include autoregressive terms in MIDAS regressions. The results obtained suggest that, in general, using MIDAS regressions con-. xvi.
(19) INTRODUCTION. xvii. tributes to increase forecast accuracy. Flexible MIDAS weighting schemes are able to exploit the information content of high-frequency series for forecasting purposes, regardless of the exact time frequency of the regressors. Another issue addressed herein is the power performance when testing for the presence of unit roots. Unit root tests typically suffer from low power in small samples, which results in not rejecting the null hypothesis as often as they should. To overcome this shortcoming, alternative tests exploiting information from covariates have been proposed. I assess whether it is possible to improve the power performance of covariate-augmented unit root tests by exploiting mixed-frequency data through the MIDAS approach. In Chapter 2 I put forward a new class of covariate-augmented Dickey-Fuller (DF) unit root tests that is able to deal with mixed-frequency data. Hansen (1995) generalised the augmented DF test to include covariates - the CADF test. The intuition is that including a weakly exogenous and stationary variable in the auxiliary test regression may lead to efficiency gains. More recently, Pesavento (2006) proposed a modified version of the CADF test, similar to the GLS generalisation of the ADF test in Elliott et al. (1996). I use MIDAS regressions to extend these two covariate-augmented DF tests to take on board mixed frequency data — the mixed-frequency CADF test (M-CADF) and the mixed-frequency CADF test with GLS demeaning/detrending (M-CADF-GLS). Alternatively to the CADF tests, Elliott and Jansson (2003) proposed a feasible point optimal unit root test in the presence of deterministic components (EJ test, hereafter), which extended the univariate results in Elliott et al. (1996). In spite of having a slightly worse size performance, the authors concluded that the EJ test outperforms the CADF test in terms of power. In Chapter 3 I go one step further and include mixed-frequency data in the EJ testing framework. Given that implementing the EJ test requires estimating VAR models, in order to plug in mixed-frequency data in the test regression I use the MIDAS vector autoregressive (VAR-MIDAS) approach. Building on Ghysels (2012), I propose an unconstrained, though parsimonious, stacked skip-sampled reduced-form VAR-MIDAS model, which is estimated using standard econometric techniques..
(20) An extensive comparison of a suite of unit root tests, with low- or mixedfrequency data, is presented. Both in Chapter 2 and Chapter 3 the results from a Monte Carlo exercise indicate that mixed-frequency tests have better power performance than low-frequency tests. Hence, covariate-augmented unit root tests also seem to benefit from exploiting high-frequency data. The gains are robust to the size of the sample, to the lag specification of the test regressions and to different combinations of time frequencies. Moreover, the EJ-family of tests tends to have a better power performance than the CADF-family of tests, either with low or mixed-frequency data. An empirical illustration using the US unemployment rate is also presented, covering all unit root tests mentioned throughout this thesis. Finally, the main results and lines for future research are summarised in the last chapter..
(21) Chapter 1 Macroeconomic Forecasting with MIDAS Abstract Techniques that allow taking full advantage of the timely releases of high-frequency data play a key role in forecasting and policymaking, especially nowadays because researchers have access to larger datasets. In this paper, I focus on the MI(xed) DA(ta) S(ampling) framework for handling different sampling frequencies and asynchronous releases of information. For forecasting quarterly euro area GDP growth using a small set of selected indicators, I run a horse race between a wide set of MIDAS regressions - original and multiplicative, with and without autoregressive terms, with and without current period information - and evaluate their performance, in terms of root mean squared forecast error, against AR and quarterly bridge models. Alternative techniques for the autoregressive augmentation are presented and discussed. Different combinations of variables, through forecast pooling and multi-variable regressions, and different time frequencies are also considered. The results obtained suggest that, in general, using MIDAS regressions contributes to increase forecast accuracy. JEL Classification: C53, E37. Keywords: MIDAS regressions, High-frequency data, Forecasting.. 1.1. Introduction. Nowadays researchers have access to more ‘comprehensive’ datasets. Not only the number of variables available has increased significantly, but data are also released on a more frequent and timely basis. However, some key macroeconomic 1.
(22) CHAPTER 1. MACROECONOMIC FORECASTING WITH MIDAS indicators are released with a significant time delay and at low frequencies (e.g., national account aggregates are typically published at quarterly frequency and with a delay of six weeks in the case of euro area GDP flash estimate and four weeks in the case of US GDP advance estimate). Therefore, forecasting models are usually set up with all variables sampled at the same frequency (the lower frequency), not capturing directly the timely releases of higher frequency data (e.g., industrial production, retail turnover and confidence indicators on a monthly basis, and stock prices, oil prices and interest rates on a daily basis). Taking into account timely releases of high frequency data is quite relevant for forecasting purposes mainly for two reasons. First, forecast accuracy is not invariant to the data frequency used (see Marcellino, 1999, for a discussion on the effects of temporal aggregation on several time series properties). Second, current period estimates (i.e. nowcasts) can benefit from within-period releases of high-frequency information. For instance, consider the release of the euro area GDP flash estimate for the fourth quarter of 2010. This figure is only available in mid-February of 2011, but until then several daily, weekly and monthly indicators relative to the fourth quarter of 2010 have been released, though not all at the same time. Results from previous studies suggest that estimates that include current period information relative to high frequency series outperform purely low frequency estimates (see, among others, R¨ unstler et al., 2009, for euro area and individual countries GDP estimates). Nowadays, this is even more relevant because the regular need for estimates on the current state of the economy in order to support policy decisions is more pressing. In this paper, I focus on the MI(xed) DA(ta) S(ampling) framework, proposed by Ghysels et al. (2004) for handling different sampling frequencies and asynchronous releases of information. Inspired in the distributed lag models, the MIDAS regressions are very flexible, being able to account for different frequencies, different aggregation polynomials and different forecast horizons (for a brief overview of the main topics related with MIDAS regressions see Andreou et al., 2011). MIDAS regressions were originally associated with empirical applications to financial series, focusing on volatility predictions; see Ghysels et al. (2004, 2006, 2007). However, MIDAS regressions have also been used in typical macroeconomic. 2.
(23) CHAPTER 1. MACROECONOMIC FORECASTING WITH MIDAS forecasting applications (see, among others, Clements and Galv˜ao, 2008 and Bai et al., 2013 for US GDP growth, Armesto et al., 2009 for the predictive content of the Beige Book, Kuzin et al., 2011 for euro area GDP, Marcellino and Schumacher, 2010 for German GDP, Monteforte and Moretti, 2013 for euro area inflation, and Asimakopoulos et al., 2013 for fiscal variables of a set of European countries). Over the years other techniques have been used to combine data with different frequencies and exploit timely releases of high frequency data for improving forecast accuracy. Some authors suggested interpolating the lower frequency data in order to specify a model in the higher frequency (see, for example, Liu, 1969, Liu and Hwa, 1974 and Engle et al., 1989). Alternative approaches include pooling forecasts from two models (e.g., a quarterly model and a monthly model) as in Corrado and Greene (1988), Howrey et al. (1991), Donihue and Howrey (1992), Miller and Chin (1996), Stark (2000) and Ruey-Wan and Chung-Hua (1996). In a slightly different vein, Klein and Sojo (1989), Parigi and Schlitzer (1995), Ingenito and Trehan (1996), Pain and S´edillot (2005), Zheng and Rossiter (2006) and Golinelli and Parigi (2007) used bridge models to link quarterly series with monthly indicators aggregated quarterly. Within a single-model framework, alternative contributions were made by Rathjens and Robins (1993), Koenig et al. (2003) and Abeysinghe (1998). Also among the model-driven (dis)aggregation techniques, and assuming the higher frequency observations as missing, there is a vast literature on estimating such missing observations relying on state-space interpretations and Kalman filtering (see, for example, Howrey, 1991, Casals et al., 2009, Zadrozny, 1990, Mittnik and Zadrozny, 2004 and Hyung and Granger, 2008). For large data sets, factor models can also be used to address the question of forecasting using mixedfrequency data (see Mariano and Murasawa, 2003, Nunes, 2005 and Proietti, 2011, among others). These models can also deal with the existence of asynchronous release schedules of high frequency series, which implies unbalanced panels with different patterns of missing values in the end of the sample - the so-called raggededge problem (see Altissimo et al., 2010, Doz et al., 2012 and Pinheiro et al., 2013 for alternative techniques). In practice, regardless of the modelling approach used, there is not a theoretical. 3.
(24) CHAPTER 1. MACROECONOMIC FORECASTING WITH MIDAS answer to the question on whether using high frequency data improves forecast accuracy over traditional low-frequency models and how that information should be used. Evidence on the improvement of forecast accuracy derived from using disaggregate series is not straightforward when the specification and the parameters of the model are no longer assumed to be known. Results in L¨ utkepohl (1984) suggest that the forecasts from the aggregated process will be superior to the aggregated forecasts from the disaggregated process for long forecast horizons. Moreover, using data with higher frequency does not necessarily mean using more information (Granger, 1998). For instance, let us consider data observed at daily and quarterly frequencies. On the one hand, daily data may contain useful information for improving the accuracy of quarterly forecasts. Nevertheless, on the other hand, daily data may be too noisy and the signals conveyed by within-quarter releases should be downplayed. In general, empirical results suggest that taking into account timely releases of information tend to contribute to the increase of the nowcast and forecast accuracy. Moreover, when compared with other techniques, the MIDAS approach is rather appealing because it is a simple, flexible and potentially parsimonious single-regression framework. For example, Bai et al. (2013) and Kuzin et al. (2011) concluded that MIDAS regressions outperform the state space approach, particularly in the short run. Moreover, compared to factor models, small-scale MIDAS regressions are easier to interpret. Given that empirical exercises play a crucial role in the assessment of the best forecasting practices, the aim of this paper is to provide some useful insights on how to combine different time frequencies for nowcasting and forecasting using the MIDAS framework. The contribution of this paper to the existing literature is twofold. Firstly, for forecasting quarterly euro area GDP growth, I run a horse race between an extended set of MIDAS regressions and evaluate their performance, in terms of root mean squared forecast error (RMSFE), against AR and other traditional quarterly models. Secondly, I address the issue of autoregressive augmentation, discussing alternative perspectives on the implications of introducing autoregressive terms in MIDAS regression. Ghysels et al. (2007) noted that including these terms led to discontinuities in the impulse response function of the. 4.
(25) CHAPTER 1. MACROECONOMIC FORECASTING WITH MIDAS regressors on the variable of interest. To rule out these discontinuities, Clements and Galv˜ao (2008) proposed imposing a common factor restriction on the autoregressive dynamics. In this paper, I question the meaning of these discontinuities for the relevant impulse response function and assess alternatives for taking into account autoregressive dynamics in MIDAS regressions, namely by not imposing a common factor and by using multiplicative and balanced autoregressive MIDAS regressions. As the evidence in favour of using large information sets is not clear-cut (see, for example, Banerjee and Marcellino, 2006), in this paper I focus on a small set of selected indicators, comparing the forecasting performance of single indicator models with forecast pooling and multiple variable models. In addition to different combinations of variables, different time frequencies are also considered. In particular, the possibility of including in the same model different high-frequency indicators, current period information and different time frequencies enables the assessment of whether there is a marginal gain from adding higher frequency data. This paper also addresses the question on whether daily data is still useful if timely monthly information is included in the model. Moreover, for a given indicator, the best frequency for forecasting purposes - lowest, highest or something in between - is assessed. The forecasts are obtained through a recursive out-of-sample exercise, which takes into account the ragged-edges of the high-frequency data and the publication delay of GDP. This pseudo real-time exercise mimics the release pattern of the indicators as they become available in real-time situations. To assess whether the gains in terms of forecast accuracy from taking on board high-frequency data are short-lived, past high-frequency data is combined to obtain nowcasts (current period forecasts) and direct forecast for different horizons, up to 4 quarters ahead. The remainder of this paper is organised as follows. Section 1.2 presents a brief description of the literature on mixed-frequency data. Section 1.3 describes the main topics related with MIDAS modelling and discusses in detail the different techniques for the autoregressive augmentation of MIDAS regressions. The design of the now- and forecasting exercise is presented in Section 1.4, while Section 1.5 focuses on the results. Finally, Section 1.6 concludes.. 5.
(26) CHAPTER 1. MACROECONOMIC FORECASTING WITH MIDAS. 1.2. Overview of the literature. On the wake of the improvement of data collection and treatment techniques, richer high-frequency datasets represent an opportunity for their users, especially those concerned with improving forecast accuracy, whether they are economic researchers or policymakers. Intuitively, one can put it this way - having larger data sets is an advantage simply because more information is better than less information. The case of datasets comprising a large number of series has been widely discussed in the popular strand of literature on factor models (see, among other, Stock and Watson, 2002, Forni et al., 2000 and Forni et al., 2005). Regarding time frequencies, some theoretical results support the idea that when confronted with the choice between using higher frequency series or using their temporal aggregates one should choose the disaggregate series. For example, Geweke (1978) showed that as the interval between observations goes to zero the discrete time approximation will be closer to the underlying continuous time model, which means that increased temporal disaggregation may help reducing the discrete time aggregation bias. Moreover, within discrete time models, L¨ utkepohl (1986) showed that, when the data generation process is a known vector ARMA, using the disaggregate series for forecasting purposes is at least as efficient, in terms of mean squared error, as using the temporally aggregated series, even if one is primarily interested in the less frequent series. However, using higher frequency data entails important challenges. Evidence on the improvement of forecast accuracy derived from using disaggregate series is not so clear-cut when the specification and the parameters of the model are no longer assumed to be known. In this case, the results in L¨ utkepohl (1984) suggest that forecasts drawn from the model for the aggregated series will be superior to aggregating forecasts from the model for the disaggregated series for long forecast horizons. In practice, although using data at a higher frequency provides more observations, it is not clear that it also provides more information (Granger, 1998). Furthermore, as not all variables are available at higher frequencies, handling mixed frequency data is not straightforward. Typically, to deal with this situation one converts the high frequency data, through temporal aggregation or systematic sampling, so that all variables in the database have the same (lower) temporal 6.
(27) CHAPTER 1. MACROECONOMIC FORECASTING WITH MIDAS frequency. However, this approach may not only lead to information losses and changes in causality relations in the case of temporal aggregation (see Weiss, 1984), but may also defeat the purpose of using readily available variables. Some authors have also suggested interpolating the lower frequency data in order to specify a model in the higher frequency (see, for example, Liu, 1969, Liu and Hwa, 1974 and Engle et al., 1989). For interpolating the low frequency data different techniques can be used, such as those suggested by Chow and Lin (1971), Fernandez (1981), Litterman (1983), and more recently by Santos Silva and Cardoso (2001). Additionally, Proietti (2006) and G´omez and Aparicio-P´erez (2009) used the state-space framework, while Angelini et al. (2006) considered the factor model technique for interpolation and backdating. Other alternative approaches have been used over the years. Recognising that monthly information has long been used to try to improve quarterly forecasts based on judgemental adjustments (informal methods), Corrado and Greene (1988) discussed a systematic way of pooling forecasts, based on add-factors, in order to improve the predictive accuracy of quarterly macroeconomic models using monthly information, making this process more systematic and transparent. While Corrado and Greene (1988) aimed at improving the forecasting performance of the quarterly Federal Reserve Board model, Howrey et al. (1991), Howrey (1991) and Donihue and Howrey (1992) applied a similar procedure to the Michigan Quarterly Econometric Model (MQEM) for the US economy. Combining forecasts from two models - a quarterly model and a monthly model - in order to maximise forecast accuracy was also considered by Miller and Chin (1996), Stark (2000) and RueyWan and Chung-Hua (1996). In a slightly different vein, Klein and Sojo (1989), Parigi and Schlitzer (1995), Ingenito and Trehan (1996), Pain and S´edillot (2005), Zheng and Rossiter (2006) and Golinelli and Parigi (2007) used bridge models to link quarterly series with monthly indicators aggregated quarterly. In order to have a full quarter, for the months that have not yet been released the quarterly aggregates of the monthly series are obtained by extrapolation through simple univariate time series models (for example, ARIMA models). So far, all the techniques mentioned always considered separate models for different frequencies. Now, suppose the aim is the same as before - using monthly. 7.
(28) CHAPTER 1. MACROECONOMIC FORECASTING WITH MIDAS information for improving the forecast accuracy of a quarterly model, but, instead of using different models, one wants to combine both time frequencies into a single model. Rathjens and Robins (1993) proposed an approach, which takes into account within-quarter information. Instead of obtaining quarterly estimates by simply aggregating monthly data (whether observed or forecast), these authors suggested adding a new data series to the low frequency model, associated with the dependent variable, defined as the difference between the third month of the quarter and the simple average of the quarter. This new series does not allow incorporating current quarter information, only information regarding movements within the previous quarters. Their results point to the existence of significant improvements on forecast accuracy from using the within-quarter variable, in the case of one-step ahead forecasts. However, this approach as some caveats: (i) it cannot be implemented if the variable of interest is not observed at both low and high time frequency; (ii) multi-step ahead forecasting is rather complex, as it requires specifying a model for the added variable; and, (iii) as within-quarter developments may be difficult to model, the gains from including this information in forecasting models may be short-lived. Koenig et al. (2003) proposed a similar approach of exploiting monthly data, also inspired on the fact that lower frequency rates of change are a weighted combination of higher frequency rates of change. The authors regressed quarterly changes in real GDP on a constant and five lagged month-on-month changes of the monthly indicators considered. No autoregressive terms were added and the coefficients were estimated unrestrictedly. If contemporaneous correlation between the variables is relevant, these models can only be estimated when values for all months in the quarter are already known. Alternatively, Abeysinghe (1998) started with a distributed lag model defined at the higher frequency, which was subsequently multiplied by a specific lag polynomial in order to convert the fractional lags of the dependent variable (not observed in the low frequency variables) into integer lags. Implicitly, the resulting lag structure gives more weight to the more recent high frequency observations. With this technique, adding too many variables has important costs in terms of degrees of freedom. Moreover, if the contemporaneous correlation between current. 8.
(29) CHAPTER 1. MACROECONOMIC FORECASTING WITH MIDAS quarter dependent and independent variables is significant, then the model can be estimated only after the release of data for the third month of the quarter (or monthly forecasts have to be incorporated). Considering data for Singapore’s GDP and external trade, the author concluded that the quarterly model with monthly data improved forecast accuracy in terms of root mean squared errors over an univariate benchmark and a quarterly model, but the gains were marginal. As noted by Abeysinghe and Tay (2000), the above-mentioned technique can be applied to both stock and flow variables. While for stock variables the interpretation is direct, for flow variables an aggregation polynomial has to be added. In the case of flow variables, combining the aggregation of the variables in the model (including the error variable) with the lag structure (lagged terms of the dependent variable) generates an autocorrelation problem, as mentioned by Abeysinghe and Tay (2000) and Abeysinghe (2000). To solve this problem, the use of instrumental variables is suggested but the results are not reported. Also among the model-driven (dis)aggregation techniques, and assuming the higher frequency observations as missing, there is a vast literature on estimating such missing observations relying on state-space interpretations and Kalman filtering (Howrey, 1991). This technique uses the serial dependence of the data in order to estimate conditional expectations of the missing observations, delivering as a by-product estimates for the unobserved values. Nevertheless, this approach entails some difficulties. In particular, state space models are typically larger and more complex models, which require the explicit specification of the dynamics of the series involved and include a large number of parameters. Casals et al. (2009) used this approach to disaggregate and forecast annual series of value added by industry in Spain, using as indicator the quarterly series of the industrial production index. Zadrozny (1990) also used the Kalman filter to estimate a multivariate ARMA model for obtaining forecasts for quarterly US GNP using monthly observations on total employment. The author concluded that this approach has a better forecasting performance in the short-run than traditional models, such as VAR models, estimated using low frequencies. Moreover, Mittnik and Zadrozny (2004) obtained similar results for German GDP. For forecasting quarterly US GDP using monthly industrial production series, Hyung and Granger (2008) also casted an. 9.
(30) CHAPTER 1. MACROECONOMIC FORECASTING WITH MIDAS ARMA model into state-space representation and estimated it, using the Kalman filter. This model was named by the authors as linked-ARMA model. According to their results, the improvements in terms of forecast accuracy of the linked-ARMA model over a traditional quarterly model and the Rathjens and Robins’ (1993) approach are not significant. However, using within-current-quarter information clearly improves forecast accuracy and the linked-ARMA model significantly outperforms the alternatives considered. Factor models can also be used for forecasting using mixed-frequency data. For example, as in Mariano and Murasawa (2003), Nunes (2005) extended the Stock and Watson (1989) factor model by including indicators available at different frequencies and concluded that this approach delivers better forecasting performance than AR(1) models. For the euro area, Proietti (2011) estimated a large scale factor model combining monthly series with quarterly national accounts series. When combining large amounts of high frequency information and estimating factor models, the existence of asynchronous release schedules of high frequency series implies unbalanced panels with different patterns of missing values in the end of the sample (the so-called ragged-edge problem). To tackle this issue, different techniques for estimating the factors have been used, namely realigning the time series in order to obtain a balanced panel (Altissimo et al., 2010), using the Expectation-Maximisation (EM) algorithm within a static principal component analysis Stock and Watson (2002) or in a dynamic factor model framework (see, among others, Doz et al., 2012 and Pinheiro et al., 2013). In general, the results point to an increase in nowcast and forecast accuracy from introducing within-period information (see, for example, Giannone et al., 2008).. 1.3. MIDAS modelling. After presenting some theoretical motivation and notation (Section 1.3.1), the MIDAS approach is discussed in Section 1.3.2. A detailed description of the different weighting schemes underlying MIDAS regressions is presented in Section 1.3.2. Finally, in Section 1.3.3 alternative methods for introducing autoregressive terms in MIDAS regressions are reviewed and a new perspective on this issue is proposed.. 10.
(31) CHAPTER 1. MACROECONOMIC FORECASTING WITH MIDAS. 1.3.1. Background. Consider the traditional low frequency regression, Yt+h = α + βQt + εt+h. (1.1). where h denotes the forecast horizon (when h = 0 the model delivers nowcasts) and both Yt+h and Qt are sampled at a low frequency, e.g., quarterly. All the parameters of the model depend on the forecast horizon and forecasts are computed directly, i.e., no additional forecasts of the explanatory variables are needed in order to obtain forecasts for the variable of interest. Equation 1.1 can be extended to include lags of the Y and Q variables, as well as additional regressors and respective lags, as follows G(L)Yt+h = α +. N X. βi (L)Qi,t + εt+h. (1.2). i=1. where G(L) and βi (L) are finite order lag polynomials, L is the conventional lag operator and N is the number of regressors. This equation is a typical autoregressive distributed lag (ADL) regression. Now, assume that Yt+h and Qt are temporal aggregates of higher frequency disaggregated series, e.g., monthly series (yt+h and xt , respectively). For each lowfrequency (quarterly) observation of Yt+h and Qt there are m (3) observations of the high-frequency (monthly) yt+h and xt series. The low-frequency variables are characterised by an aggregation scheme Γ(L1/m ), which can be one of the following: 1. stock variable Yt =. h. 1 0 0. i. (3). yt. . (3) × yt−1/3 (3) yt−2/3. 2. flow variable. 11. (1.3).
(32) CHAPTER 1. MACROECONOMIC FORECASTING WITH MIDAS (a) sum (3). Yt =. h. 1 1 1. i. . yt. (3) × yt−1/3 (3) yt−2/3. (1.4). (b) equal-weight average Yt =. h. 1/3 1/3 1/3. i. (3). . yt. (3) × yt−1/3 (3) yt−2/3 (3). (c) rate of change (assume that y˜t. (1.5). (3). are the levels underlying the yt. rates. of change) Yt =. (3). (3). (3) ˜t−1−(i/3) i=0 y. (3). y˜t−4/3. y˜t−1 P2. y˜t−5/3. (3) ˜t−1−(i/3) i=0 y. P2. (3) ˜t−1−(i/3) i=0 y. P2. . (3). yt. . (3) × yt−1/3 (1.6) (3) yt−2/3. (d) unrestricted linear combination Yt =. (3). where yt that. (3) yt. h. γ0 γ1 γ2. i. (3). yt. . (3) × yt−1/3 (3) yt−2/3. (1.7). is a skip-sampled version of the high-frequency yt series, meaning. and Yt have the same number of quarterly observations.. In time series analysis, observed time series are often temporal aggregates of unobserved disaggregated series. As before, assume for instance that Qt is observed monthly (xt ), while Yt+h is only observed quarterly - meaning that yt+h is not observed (flow variable) or has missing observations (stock variable). Although regression analysis would ideally try to approximate the original data generating. 12.
(33) CHAPTER 1. MACROECONOMIC FORECASTING WITH MIDAS process (DGP) by using high-frequency samples, as in the following equation. g(L. 1/m. )yt+h = a +. N X. bi (L1/m )xi,t + et+h. (1.8). i=1. where g(L1/m ) and bi (L1/m ) are finite order lag polynomials, this is not always possible. The mixed-frequency approaches suggest mixing a low-frequency dependent variable on the left-hand side with high-frequency regressors on the right-hand side. Assuming that high-frequency yt+h would be well represented by equation 1.8, there is a φ(L1/m ) polynomial such that h(L) = g(L1/m )φ(L1/m ). Multiplying both sides of equation 1.8 by φ(L1/m ) and the aggregation scheme Γ(L1/m ) one obtains φ(L. 1/m. 1/m. )g(L. )Γ(L. 1/m. N X. )yt+h = a ¨+. bi (L1/m )φ(L1/m )Γ(L1/m )xi,t. i=1 1/m. + φ(L h(L)Yt+h = a ¨+. )Γ(L1/m )et+h. N X. bi (L1/m )zi,t + ξt+h. (1.9). i=1. where a ¨ = φ(L1/m )Γ(L1/m )a, zi,t = φ(L1/m )Γ(L1/m )xi,t and ξt+h = φ(L1/m ) Γ(L1/m )et+h for t = m, 2m, 3m, .... Equation 1.9 is the exact mixed-frequency model associated with the high-frequency model in equation 1.8, relating the lowfrequency Yt+h dependent variable with the high-frequency xi,t regressors. As discussed in Wei and Stram, 1990, Marcellino, 1998 and Marcellino, 1999, among others, in general it is not possible to uniquely identify φ(L1/m ) in this single-equation framework and, so, neither the g(L1/m ) and bi (L1/m ) polynomials. This means that, in general, one can only approximate the mixed-frequency model, as follows ˜ h(L)Y ˜+ t+h = a. N X. ˜bi (L1/m )x(3) + ut+h i,t. (1.10). i=1 (3). where xi,t is the skip-sampled version of the high-frequency xi,t and the orders 13.
(34) CHAPTER 1. MACROECONOMIC FORECASTING WITH MIDAS ˜ of the polynomials h(L) and ˜bi (L1/m ) are data-driven (e.g., selected from information criteria). Furthermore, since one cannot recover the high-frequency g(L1/m ) and bi (L1/m ) polynomials, it is also not possible to identify the high-frequency impulse response function of xi,t on yt . Hence, the approximate mixed-frequency (3). model implies an observable quarterly impulse response function of xi,t on Yt . Underlying this observable impulse response function are the latent monthly impulse response functions of xi,t on yt .. 1.3.2. From the approximate mixed-frequency regression to MIDAS regressions. Equation 1.10 is a MIDAS regression. More precisely, equation 1.10 as been referred to in the literature as an unrestricted MIDAS regression; see e.g. Marcellino and Schumacher (2010), Foroni and Marcellino (2012) and Foroni et al. (2011). This regression is one of the particular cases covered by the general MIDAS framework. Introduced by Ghysels et al. (2004) and initially presented in Ghysels et al. (2006) or Ghysels et al. (2007), the MIDAS approach provides simple, reduced-form models to approximate more elaborate, though unknown, high-frequency models. Original MIDAS regressions assume that the coefficients of ˜bi (L1/m ) in equation 1.10 are captured by a known weight function B(j; θ), (3). Yt+h = β0 + β1 B(L1/m ; θ)xt + ut+h where B(L1/m ; θ) =. PJ. j=0. (1.11). B(j; θ)Lj/m is a polynomial of length J in the L1/m. operator, B(j; θ) represents the weighting scheme used for the aggregation, which is assumed to be normalised to sum to 1, β1 is the slope coefficient, β0 is a constant, (m). Lj/m xt. (m). = xt−j/m and ut+h is a standard iid error term. Similarly to distributed. lag models (for further details on distributed lag models, see Dhrymes, 1972 and Griliches, 1967, among others), Ghysels et al. (2004) show that the aggregation bias also converges to zero in the MIDAS framework when m → 0. Hence, regardless of the unavailability of Yt+h at a higher frequency, using high-frequency regressors can mitigate the aggregation bias mentioned by Sims (1971) and Geweke (1978). Although the order of the polynomial B(L1/m ; θ), i.e. J, is potentially infinite, 14.
(35) CHAPTER 1. MACROECONOMIC FORECASTING WITH MIDAS some restrictions must be imposed for the sake of tractability and, thus, the choice of J is an important step in the model specification (see, for example, Judge et al., 1985). Typically, information criteria, such as AIC and BIC, are used for model specification purposes. The restrictions result from the need of a balance between the gains in terms of additional information (more lags) and the costs of parameter proliferation. Nevertheless, in the original MIDAS regressions, the coefficients of B(L1/m ; θ) are captured by a known weight function B(j; θ), which depends on a few parameters summarised in vector θ. Thus, in this case, MIDAS regressions are tightly parameterised. Regardless of the choice of a specific weight function (discussed in more detail in Section 1.3.2 below), MIDAS regressions allow for more flexible, data-driven weighting schemes than commonly used schemes, such as sum or equal-weight average (equations 1.4 and 1.5). For example, as Andreou et al. (2010) analytically demonstrate, traditional equal-weight aggregation is nested in MIDAS regressions, i.e., Yt+h. J X 1 (m) = β0 + β1 Qt + β1 (B(j; θ) − )∆J−(j+1) xt−j/m + ut+h J j=0. (1.12). where ∆ = 1 − L is the difference operator. Given that alternative aggregation functions of high-frequency data imply different restrictions on the B(L1/m ; θ) polynomial, the main question is which restrictions fit the data better. Focusing on equation 1.12, in case MIDAS regressions outperform the traditional approach, this implies that averaging may not be the aggregation method that maximises the ability of a high-frequency variable to predict a variable only available at a lower sampling frequency, meaning that B(j; θ) 6= (1/J) and, so, the coefficients (B(j; θ) − J1 ) do not cancel out. Hence, MIDAS regressions allow for more flexible weighting structures than traditional low-frequency models and also tend to be more parsimonious. Moreover, the MIDAS framework can easily accommodate the timely releases of high(m). frequency data. In (1.11), where Yt+h and xt. are contemporaneously related,. it is assumed that all high-frequency observations over the current low-frequency period are known. Considering quarterly and monthly data, this means that the 15.
(36) CHAPTER 1. MACROECONOMIC FORECASTING WITH MIDAS three months in the quarter are already available. If instead of a full-quarter, say, only the first month is available, then the MIDAS regression can be rewritten as (3). Yt+h = β0 + β1 B(L1/3 ; θ)xt−2/3 + ut+h .. (1.13). Alternatively, the current-period information can be associated with a different β coefficient. Furthermore, MIDAS regressions can be extended to accommodate different (high) frequencies and additional indicators and, in most cases, without requiring many more parameters to be estimated. Thus, a more general version of MIDAS regressions, which can handle multiple regressors with different time frequencies, is as follows: Yt+h = β0 +. N X. (m ). βn Bn (L1/mn ; θ)xt,nn + ut+h. (1.14). n=1. where N refers to the number of indicators and mn to the time frequency of each indicator. Moreover, different polynomials Bn (L1/mn ; θ) for each regressor can also be considered. Although it is possible to have multivariate MIDAS regressions, the number of regressors included is necessarily limited. However, the MIDAS framework can still be used if one is interested in exploiting large information sets. Combining two important strands of the literature on forecasting using high frequency indicators, Marcellino and Schumacher (2010) merge factor models with MIDAS, introducing the Factor-MIDAS approach, which is applied to nowcast German GDP quarterly developments. Instead of focusing on a single regressor, or a small group of regressors, the authors extracted factors from a large set of variables, and compared the performance of different techniques for extracting factors in the presence of ragged-edge data. Then, the monthly factors were plugged into MIDAS regressions to estimate current quarter GDP. This approach is compared with an integrated state-space approach, as used in Altissimo et al. (2010), and with unrestricted MIDAS regressions. The results presented in Marcellino and Schumacher (2010) suggest that MIDAS regressions perform well when compared with state-space models.. 16.
(37) CHAPTER 1. MACROECONOMIC FORECASTING WITH MIDAS Weighting schemes Ghysels et al. (2007) considered two alternatives for the weighting function, both assuming that the weights are determined by a few hyperparameters θ: the exponential Almon lag e(θ1 j+θ2 j. B(j; θ1 , θ2 ) = PJ. i=1. 2). (1.15). e(θ1 i+θ2 i2 ). and the beta polynomial f ( j , θ1 , θ2 ) B(j; θ1 , θ2 ) = PJ J i i=1 f ( J , θ1 , θ2 ). (1.16). where f (q, θ1 , θ2 ) = (q θ1 −1 (1−q)θ2 −1 Γ(θ1 +θ2 ))/(Γ(θ1 )Γ(θ2 )) and Γ(θ) =. R∞ 0. e−k. k θ−1 dk. Given that exponential Almon and beta polynomials have nonlinear functional specifications, in both cases MIDAS regressions have to be estimated using nonlinear methods, namely nonlinear least squares. Chen and Ghysels (2010) discussed a multiplicative MIDAS framework, which is closer to traditional aggregation. Instead of aggregating all lags in the high frequency variable to a single aggregate, multiplicative MIDAS regressions include m-aggregates of high-frequency data and their lags, Yt+h = β0 +. p X. βi+1 xmult t−i + ut+h. (1.17). i=1. where xmult = t. Pm−1 j=0. (m). B(j; θ)Lj/m xt . In case many lags need to be included, a. restricted version of the model can be used, where the coefficients associated with the m-aggregates and their lags (βi ) are also determined by a given polynomial, such as the exponential Almon or the beta polynomial. The multiplicative MIDAS model can also include current-period information, but in this case these data are necessarily associated with a different coefficient. Bai et al. (2013) demonstrated that, in ideal circumstances (namely, in population and assuming correctly specified models), multiplicative MIDAS regressions can match exactly the steady state Kalman filter. In practice, when parameter. 17.
(38) CHAPTER 1. MACROECONOMIC FORECASTING WITH MIDAS estimation and model specification errors also play a part, empirical exercises are needed in order to assess the forecasting performance of MIDAS vs. state space models. To forecast quarterly US GDP growth, Bai et al. (2013) showed that MIDAS regressions outperform the state space approach in short-term forecast horizons. Moreover, within MIDAS regressions, the original and multiplicative regressions have similar performances, neither one clearly dominating the other. Similarly, for euro area GDP, Kuzin et al. (2011) compared the forecasting performance of MIDAS regressions with mixed frequency VAR models, as proposed by Zadrozny (1988) and Mittnik and Zadrozny (2004), using a set of about 20 monthly indicators. These authors also concluded that MIDAS regressions perform better in shorter horizons, while state space models tend to perform better in longer horizons. Inspired by the Heterogeneous Autoregressive models (HAR) in Corsi (2009), Forsberg and Ghysels (2007) considered an alternative weighting scheme, the socalled step functions. Instead of using a known function determined by a few parameters, step functions correspond to partial sums of the high-frequency vari(m). able xt , as follows: Yt+h = β0 +. S X. βs zs(m) + ut+h. s=1. s zs(m) = x(m) Ij∈[a s−1 ,as ]. s Ij∈[a s−1 ,as ]. 1, a s−1 < j ≤ as = 0, otherwise. (1.18). where a0 = 1 < a1 < . . . < aS = J, S is the number of steps, with S <= J, s Ij∈[a s−1 ,as ] (m). and x. is a (J × 1) vector (there are as many I s vectors as the number of steps), (m). is a matrix with the J lags of xt . For example, assume that x(m). represents 30 lags (a full month) of a daily series. Additionally, assume that these data are aggregated through step functions, using 3 steps - [1 − 10], [11 − 20] and [21 − 30]. Then, s = 1, 2 or 3. For s = 1, I s is a vector with 30 observations, 18.
(39) CHAPTER 1. MACROECONOMIC FORECASTING WITH MIDAS which equals 1 for j = 1 to 10 and 0 for j = 11 to 30. As there is a βs coefficient for each step function, the more steps the less parsimonious the model will be. Although losing in terms of parsimony for the other two weighting schemes, the step functions are easier to estimate. In this case, ordinary least squares (OLS) can be used for estimating MIDAS regressions because step functions are linear. There are two additional weighting schemes that also lead to regressions that can be estimated by OLS. The first one is the traditional Almon lag polynomial. This aggregation scheme assumes that J lag weights can be related to d linearly estimable underlying parameters, with d < J, as follows: B(j; θi ) =. d X. θi j i. (1.19). i=1. where j = 1, . . . , J and i = 1, . . . , d. The second is the aggregation scheme underlying the above-mentioned unrestricted MIDAS regressions. The unrestricted MIDAS regressions are nested in the MIDAS regression with step functions, with a different ‘step’ for each highfrequency lag, such as (m). Yt+h = β0 + Bu (L1/m )xt = β0 +. J X. + ut+h (m). βj+1 Lj/m xt. + ut+h. j=0 (m). = β 0 + β 1 xt. (m). (m). + β2 xt−1/m + . . . + βJ+1 xt−J/m + ut+h .. (1.20). When the difference between the low and the high frequency is large, estimating a regression such as (1.20) can involve a large number of parameters. In this case, large differences in sampling frequencies between the variables considered are readily penalised in terms of parsimony. For instance, if Yt+h is sampled quarterly and xt refers to daily data, then estimating a mixed-frequency regression can easily involve estimating more than 60 parameters. The unrestricted MIDAS regressions are better able to deal with mixing quarterly and monthly data (as in Marcellino and Schumacher, 2010, for example) for which the parameter proliferation shortcoming is less stringent, than mixing quarterly with weekly, daily or intra-daily 19.
(40) CHAPTER 1. MACROECONOMIC FORECASTING WITH MIDAS data. Regarding its empirical performance, for nowcasting German GDP quarterly developments, Marcellino and Schumacher (2010) found that there are no big differences between alternative - original and unrestricted - MIDAS approaches. Using data for both the euro area and the US, Foroni et al. (2011) concluded that unrestricted MIDAS regression deliver a better in-sample performance than original MIDAS regressions when m is small. The results for the out-of-sample GDP forecasting exercise were not clear-cut and neither model globally dominated the other.. 1.3.3. Autoregressive augmentation of MIDAS regressions. Originally, the MIDAS approach was associated with empirical applications to financial series, focusing on volatility predictions; see Ghysels et al. (2004, 2006, 2007). However, MIDAS regressions have also been used in macroeconomic applications. For example, Clements and Galv˜ao (2008) and Bai et al. (2013) assessed whether the MIDAS framework is a good tool for forecasting output growth in the US. Moreover, Armesto et al. (2009) used MIDAS regressions to assess the predictive content of the Beige Book (US Federal Reserve System regional highfrequency information). Monteforte and Moretti (2013) used MIDAS regressions for forecasting monthly euro area inflation (current and one-month ahead) resorting to a monthly index of core inflation and daily data from financial markets. The inclusion of autoregressive dynamics is an important feature of forecasting models, especially for macroeconomic applications. In this section the initial contributions to the literature regarding the introduction of autoregressive terms in MIDAS regressions are reviewed and, then, an alternative perspective on this subject is presented. For the sake of simplicity and unless otherwise stated, a first order autoregression and h = 0 are assumed. Notwithstanding, the notation can be easily extended. Initial contributions Ghysels et al. (2007) discussed the implications of introducing autoregressive terms in MIDAS regressions and suggested two possible ways of doing this, noting that. 20.
(41) CHAPTER 1. MACROECONOMIC FORECASTING WITH MIDAS both solutions had caveats. In the first case, a polynomial in L1/m is considered, (m). Yt = β0 + β1 B(L1/m ; θ)xt. + γYt−(1/m) + ut. (1.21). which implicitly assumes that Yt−(1/m) is available. This solution may not be very appealing because if high-frequency lags of Yt were available then probably it would be possible to set up a high-frequency regression. Moreover, Ghysels et al. (2007) remarked that estimating (1.21) is more challenging because including the term Yt−(1/m) forces one to deal with endogenous regressors and with instrumental variable estimation. In the second case, a polynomial in L is considered, i.e. (m). Yt = β0 + β1 B(L1/m ; θ)xt Yt = β0∗ + β1. + γYt−1 + ut. 1/m. B(L ; θ) (m) xt + u∗t (1 − γL). where B(L1/m ; θ) =. PJ. j=0. (1.22). B(j; θ)Lj/m , β0∗ = β0 /(1 − γ) and u∗t = ut /(1 − γL).. Considering the distributed lag representation, instead of a polynomial in L1/m one obtains a mixture. B(L1/m ;θ) . (1−γL). Equation 1.23 shows more clearly the shape of. the polynomial, for example assuming J = m − 1 = 2 for the sake of simplicity, we can see that (3). (3). (3). (3). (3). (3). Yt = β0∗ + β1 (B0 xt + B1 xt−1/3 + B2 xt−2/3 + γB0 xt−1 + γB1 xt−4/3 + γB2 xt−5/3 (3). (3). (3). +γ 2 B0 xt−2 + γ 2 B1 xt−7/3 + γ 2 B2 xt−8/3 + ...) + u∗t. (1.23). Ghysels et al. (2007) and Andreou et al. (2011) pointed out that the autoregressive distributed lag MIDAS regression in equation 1.22 entails an undesirable property - the. B(L1/m ;θ) (1−γL). polynomial displays geometrically declining spikes at dis-. tance m, mimicking a seasonal pattern. This autoregressive augmentation of MI(m). DAS regressions should be used if a seasonal pattern in xt. is detected (Ghysels. et al., 2007). To illustrate these so-called spikes, Figure 1.1 plots simulated sequences of the (3). coefficients associated with xt. and its lags (up to 26 lags). The solid black line. refers to equation 1.23, with J = m − 1 = 2, meaning that the high-frequency 21.
(42) CHAPTER 1. MACROECONOMIC FORECASTING WITH MIDAS (a) High γ. (b) Low γ. 0.7. 0.7. 0.6. 0.6. 0.5. 0.5. 0.4. 0.4. 0.3. 0.3. 0.2. 0.2. (c) Equal weights 0.45 0.40 0.35 0.30 0.25 0.20 0.15. 0.1. 0.10. 0.1. 0.0 0. 2. 4 J=2. 6. 8 10 12 14 16 18 20 22 24 26 J=3. J=5. 0.05 0.00. 0.0 0. 2. 4 6 J=2. 8 10 12 14 16 18 20 22 24 26 J=3 J=5. 0. 2. 4. 6. 8 10 12 14 16 18 20 22 24 26. J=2. J=3. (3). Figure 1.1: Simulated sequences of the coefficients associated with xt. J=5. and its lags. Note: In panels 1.1a and 1.1b the assumptions for the Bi coefficients are the following: if J = 2, B0 = 0.60, B1 = 0.25 and B2 = 0.15; if J = 3, B0 = 0.45, B1 = 0.30, B2 = 0.15 and B3 = 0.10; finally, if J = 5, B0 = 0.25, B1 = 0.40, B2 = 0.20, B3 = 0.075, B4 = 0.05 and B5P= 0.025. In J panels 1.1a and 1.1c γ = 0.7, while in panel 1.1b γ = 0.2. In all cases, β1 = 1 and i=0 Bi = 1.. terms cover a full low-frequency period (m = 3). The dashed black line represents the case where J = 3 and the solid grey line is for J = 5 (two low-frequency periods). Two alternatives for the autoregressive γ coefficient are considered: in panels 1.1a and 1.1c γ = 0.7, while in panel 1.1b γ = 0.2. Moreover, different weighting schemes are also assessed, as in Ghysels et al. (2007). Panels 1.1a and 1.1b display rapidly declining weights for J = 2, slower declining weights for J = 3 and hump-shaped weights for J = 5. Panel 1.1c plots the equal-weight case. By looking at Figure 1.1 one can see that all three simple sets of weights that try to mimic the polynomial weighting schemes display a spiky pattern and, as expected, this pattern is softened with smaller γ. Note that with unrestricted MIDAS the pattern could be even more irregular, as the weights/coefficients are estimated unrestrictedly, not obeying to a known polynomial function. In the traditional case of equal-weight schemes, the sequence of coefficients have a stepwise pattern, except when the number of high-frequency terms do not fully cover low-frequency periods (e.g. when J = 3), also exhibiting spikes. Clements and Galv˜ao (2008) suggested an alternative way of introducing autoregressive dynamics in MIDAS regressions. The authors proposed interpreting the dynamics in Yt as a common factor (Hendry and Mizon, 1978). This as-. 22.
Imagem
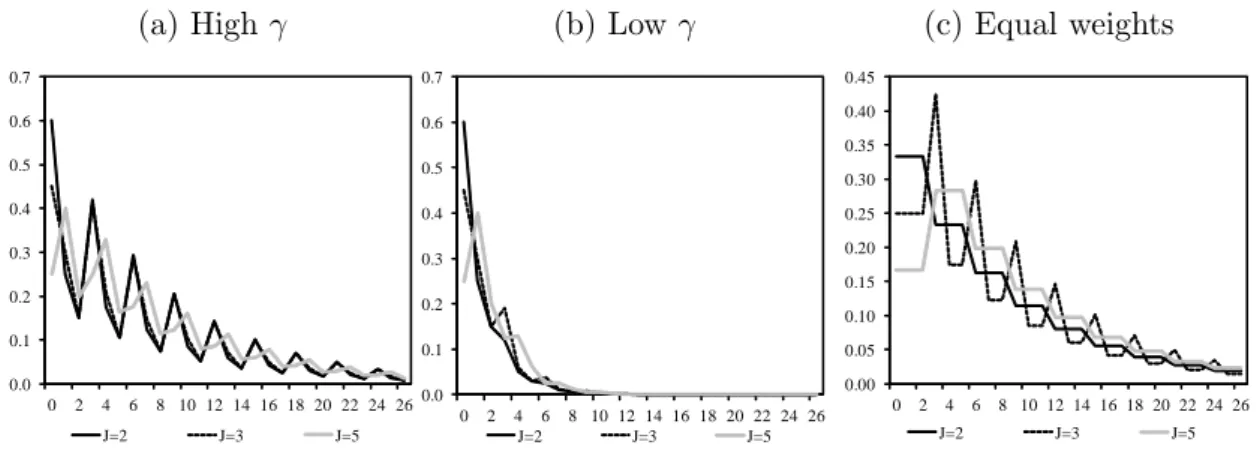
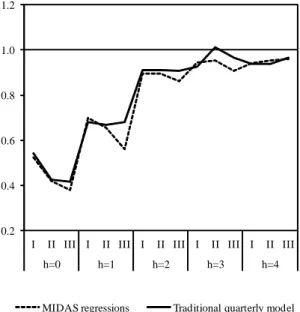
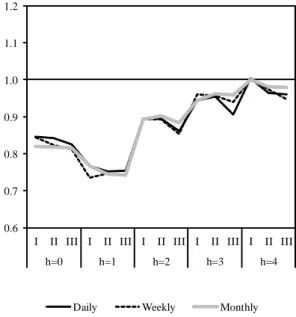
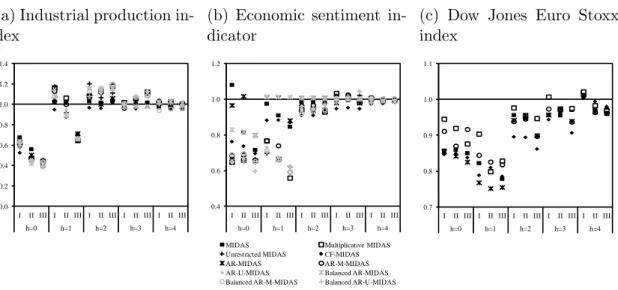
Documentos relacionados
we conducted a series of experiments trying out different percentages of collision with reaction and number of time steps and calculating the theoretical values
Specialty section: This article was submitted to Aquatic Microbiology, a section of the journal Frontiers in Microbiology Received: 30 November 2015 Accepted: 29 February
Entre eles, destacam-se os Scores de Alerta Precoce, que são Sistemas “Track and Trigger” agregados e ponderados que consistem na atribuição ponderada de scores aos
The data collected covered: demographic data and data on existing comorbidities on admission; the dose, frequency and administration method of prescribed pharmaceutics; results
Data on the sample characteristics were descriptively analyzed (mean and standard deviation) for continuous data and frequency distribution (percentage) for categorical data.
Since data on HIV testing in- tentions were not collected, we used HIV testing history to distinguish between a group with likely high testing frequency and incident HIV
Standard unit root tests have a key role in structural hypothesis
By applying a metaheuristics fuzzy model in a low-frequency data for household electricity demand forecasting, results suggested that the proposed GPU learning strategy is scalable